2024
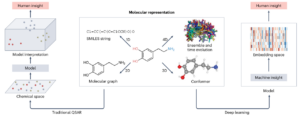
Tropsha, Alexander; Isayev, Olexandr; Varnek, Alexandre; Schneider, Gisbert; Cherkasov, Artem
Integrating QSAR modelling and deep learning in drug discovery: the emergence of deep QSAR Journal Article
In: Nat Rev Drug Discov, vol. 23, no. 2, pp. 141–155, 2024.
Abstract | Links | BibTeX | Tags: Drug Discovery, Generative AI, Review
@article{Tropsha2023,
title = {Integrating QSAR modelling and deep learning in drug discovery: the emergence of deep QSAR},
author = {Alexander Tropsha and Olexandr Isayev and Alexandre Varnek and Gisbert Schneider and Artem Cherkasov},
doi = {10.1038/s41573-023-00832-0},
year = {2024},
date = {2024-01-12},
urldate = {2024-01-12},
journal = {Nat Rev Drug Discov},
volume = {23},
number = {2},
pages = {141--155},
publisher = {Springer Science and Business Media LLC},
abstract = {Quantitative structure\textendashactivity relationship (QSAR) modelling, an approach that was introduced 60 years ago, is widely used in computer-aided drug design. In recent years, progress in artificial intelligence techniques, such as deep learning, the rapid growth of databases of molecules for virtual screening and dramatic improvements in computational power have supported the emergence of a new field of QSAR applications that we term ‘deep QSAR’. Marking a decade from the pioneering applications of deep QSAR to tasks involved in small-molecule drug discovery, we herein describe key advances in the field, including deep generative and reinforcement learning approaches in molecular design, deep learning models for synthetic planning and the application of deep QSAR models in structure-based virtual screening. We also reflect on the emergence of quantum computing, which promises to further accelerate deep QSAR applications and the need for open-source and democratized resources to support computer-aided drug design.},
keywords = {Drug Discovery, Generative AI, Review},
pubstate = {published},
tppubtype = {article}
}
2023
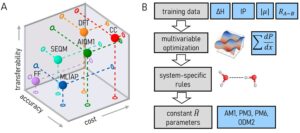
Fedik, Nikita; Nebgen, Benjamin; Lubbers, Nicholas; Barros, Kipton; Kulichenko, Maksim; Li, Ying Wai; Zubatyuk, Roman; Messerly, Richard; Isayev, Olexandr; Tretiak, Sergei
Synergy of semiempirical models and machine learning in computational chemistry Journal Article
In: J. Chem. Phys., vol. 159, no. 11, pp. 110901 , 2023.
Abstract | Links | BibTeX | Tags: Machine learning potential, Review
@article{Fedik2023,
title = {Synergy of semiempirical models and machine learning in computational chemistry},
author = {Nikita Fedik and Benjamin Nebgen and Nicholas Lubbers and Kipton Barros and Maksim Kulichenko and Ying Wai Li and Roman Zubatyuk and Richard Messerly and Olexandr Isayev and Sergei Tretiak},
doi = {10.1063/5.0151833},
year = {2023},
date = {2023-09-21},
urldate = {2023-09-21},
journal = {J. Chem. Phys.},
volume = {159},
number = {11},
pages = {110901 },
publisher = {AIP Publishing},
abstract = {Catalyzed by enormous success in the industrial sector, many research programs have been exploring data-driven, machine learning approaches. Performance can be poor when the model is extrapolated to new regions of chemical space, e.g., new bonding types, new many-body interactions. Another important limitation is the spatial locality assumption in model architecture, and this limitation cannot be overcome with larger or more diverse datasets. The outlined challenges are primarily associated with the lack of electronic structure information in surrogate models such as interatomic potentials. Given the fast development of machine learning and computational chemistry methods, we expect some limitations of surrogate models to be addressed in the near future; nevertheless spatial locality assumption will likely remain a limiting factor for their transferability. Here, we suggest focusing on an equally important effort\textemdashdesign of physics-informed models that leverage the domain knowledge and employ machine learning only as a corrective tool. In the context of material science, we will focus on semi-empirical quantum mechanics, using machine learning to predict corrections to the reduced-order Hamiltonian model parameters. The resulting models are broadly applicable, retain the speed of semiempirical chemistry, and frequently achieve accuracy on par with much more expensive ab initio calculations. These early results indicate that future work, in which machine learning and quantum chemistry methods are developed jointly, may provide the best of all worlds for chemistry applications that demand both high accuracy and high numerical efficiency.},
keywords = {Machine learning potential, Review},
pubstate = {published},
tppubtype = {article}
}
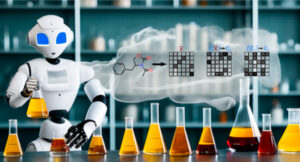
Anstine, Dylan M.; Isayev, Olexandr
Generative Models as an Emerging Paradigm in the Chemical Sciences Journal Article
In: J. Am. Chem. Soc., vol. 145, no. 16, pp. 8736–8750, 2023.
Abstract | Links | BibTeX | Tags: Drug Discovery, Generative AI, Review, RL
@article{Anstine2023b,
title = {Generative Models as an Emerging Paradigm in the Chemical Sciences},
author = {Dylan M. Anstine and Olexandr Isayev},
doi = {10.1021/jacs.2c13467},
year = {2023},
date = {2023-04-26},
urldate = {2023-04-26},
journal = {J. Am. Chem. Soc.},
volume = {145},
number = {16},
pages = {8736--8750},
publisher = {American Chemical Society (ACS)},
abstract = {Traditional computational approaches to design chemical species are limited by the need to compute properties for a vast number of candidates, e.g., by discriminative modeling. Therefore, inverse design methods aim to start from the desired property and optimize a corresponding chemical structure. From a machine learning viewpoint, the inverse design problem can be addressed through so-called generative modeling. Mathematically, discriminative models are defined by learning the probability distribution function of properties given the molecular or material structure. In contrast, a generative model seeks to exploit the joint probability of a chemical species with target characteristics. The overarching idea of generative modeling is to implement a system that produces novel compounds that are expected to have a desired set of chemical features, effectively sidestepping issues found in the forward design process. In this contribution, we overview and critically analyze popular generative algorithms like generative adversarial networks, variational autoencoders, flow, and diffusion models. We highlight key differences between each of the models, provide insights into recent success stories, and discuss outstanding challenges for realizing generative modeling discovered solutions in chemical applications.},
keywords = {Drug Discovery, Generative AI, Review, RL},
pubstate = {published},
tppubtype = {article}
}
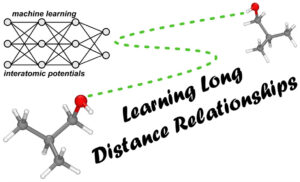
Anstine, Dylan M.; Isayev, Olexandr
Machine Learning Interatomic Potentials and Long-Range Physics Journal Article
In: J. Phys. Chem. A, vol. 127, no. 11, pp. 2417–2431, 2023, ISSN: 1520-5215.
Abstract | Links | BibTeX | Tags: AIMNet, ANI, Machine learning potential, Review
@article{Anstine2023,
title = {Machine Learning Interatomic Potentials and Long-Range Physics},
author = {Dylan M. Anstine and Olexandr Isayev},
doi = {10.1021/acs.jpca.2c06778},
issn = {1520-5215},
year = {2023},
date = {2023-03-23},
urldate = {2023-03-23},
journal = {J. Phys. Chem. A},
volume = {127},
number = {11},
pages = {2417--2431},
publisher = {American Chemical Society (ACS)},
abstract = {Advances in machine learned interatomic potentials (MLIPs), such as those using neural networks, have resulted in short-range models that can infer interaction energies with near ab initio accuracy and orders of magnitude reduced computational cost. For many atom systems, including macromolecules, biomolecules, and condensed matter, model accuracy can become reliant on the description of short- and long-range physical interactions. The latter terms can be difficult to incorporate into an MLIP framework. Recent research has produced numerous models with considerations for nonlocal electrostatic and dispersion interactions, leading to a large range of applications that can be addressed using MLIPs. In light of this, we present a Perspective focused on key methodologies and models being used where the presence of nonlocal physics and chemistry are crucial for describing system properties. The strategies covered include MLIPs augmented with dispersion corrections, electrostatics calculated with charges predicted from atomic environment descriptors, the use of self-consistency and message passing iterations to propagated nonlocal system information, and charges obtained via equilibration schemes. We aim to provide a pointed discussion to support the development of machine learning-based interatomic potentials for systems where contributions from only nearsighted terms are deficient.},
keywords = {AIMNet, ANI, Machine learning potential, Review},
pubstate = {published},
tppubtype = {article}
}
2022
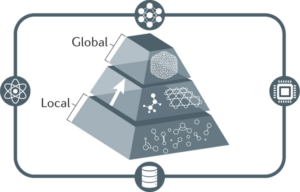
Fedik, Nikita; Zubatyuk, Roman; Kulichenko, Maksim; Lubbers, Nicholas; Smith, Justin S.; Nebgen, Benjamin; Messerly, Richard; Li, Ying Wai; Boldyrev, Alexander I.; Barros, Kipton; Isayev, Olexandr; Tretiak, Sergei
Extending machine learning beyond interatomic potentials for predicting molecular properties Journal Article
In: Nat Rev Chem, vol. 6, no. 9, pp. 653–672, 2022.
Abstract | Links | BibTeX | Tags: Machine learning potential, Review
@article{Fedik2022,
title = {Extending machine learning beyond interatomic potentials for predicting molecular properties},
author = {Nikita Fedik and Roman Zubatyuk and Maksim Kulichenko and Nicholas Lubbers and Justin S. Smith and Benjamin Nebgen and Richard Messerly and Ying Wai Li and Alexander I. Boldyrev and Kipton Barros and Olexandr Isayev and Sergei Tretiak},
doi = {10.1038/s41570-022-00416-3},
year = {2022},
date = {2022-10-14},
urldate = {2022-10-14},
journal = {Nat Rev Chem},
volume = {6},
number = {9},
pages = {653--672},
publisher = {Springer Science and Business Media LLC},
abstract = {Machine learning (ML) is becoming a method of choice for modelling complex chemical processes and materials. ML provides a surrogate model trained on a reference dataset that can be used to establish a relationship between a molecular structure and its chemical properties. This Review highlights developments in the use of ML to evaluate chemical properties such as partial atomic charges, dipole moments, spin and electron densities, and chemical bonding, as well as to obtain a reduced quantum-mechanical description. We overview several modern neural network architectures, their predictive capabilities, generality and transferability, and illustrate their applicability to various chemical properties. We emphasize that learned molecular representations resemble quantum-mechanical analogues, demonstrating the ability of the models to capture the underlying physics. We also discuss how ML models can describe non-local quantum effects. Finally, we conclude by compiling a list of available ML toolboxes, summarizing the unresolved challenges and presenting an outlook for future development. The observed trends demonstrate that this field is evolving towards physics-based models augmented by ML, which is accompanied by the development of new methods and the rapid growth of user-friendly ML frameworks for chemistry.},
keywords = {Machine learning potential, Review},
pubstate = {published},
tppubtype = {article}
}
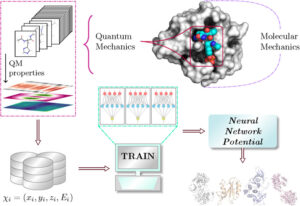
Gokcan, Hatice; Isayev, Olexandr
Learning molecular potentials with neural networks Journal Article
In: WIREs Comput Mol Sci, vol. 12, no. 2, pp. e1564, 2022.
Abstract | Links | BibTeX | Tags: ANI, Machine learning potential, Review
@article{Gokcan2021,
title = {Learning molecular potentials with neural networks},
author = {Hatice Gokcan and Olexandr Isayev},
doi = {10.1002/wcms.1564},
year = {2022},
date = {2022-07-14},
journal = {WIREs Comput Mol Sci},
volume = {12},
number = {2},
pages = {e1564},
publisher = {Wiley},
abstract = {\<jats:title\>Abstract\</jats:title\>\<jats:p\>The potential energy of molecular species and their conformers can be computed with a wide range of computational chemistry methods, from molecular mechanics to ab initio quantum chemistry. However, the proper choice of the computational approach based on computational cost and reliability of calculated energies is a dilemma, especially for large molecules. This dilemma is proved to be even more problematic for studies that require hundreds and thousands of calculations, such as drug discovery. On the other hand, driven by their pattern recognition capabilities, neural networks started to gain popularity in the computational chemistry community. During the last decade, many neural network potentials have been developed to predict a variety of chemical information of different systems. Neural network potentials are proved to predict chemical properties with accuracy comparable to quantum mechanical approaches but with the cost approaching molecular mechanics calculations. As a result, the development of more reliable, transferable, and extensible neural network potentials became an attractive field of study for researchers. In this review, we outlined an overview of the status of current neural network potentials and strategies to improve their accuracy. We provide recent examples of studies that prove the applicability of these potentials. We also discuss the capabilities and shortcomings of the current models and the challenges and future aspects of their development and applications. It is expected that this review would provide guidance for the development of neural network potentials and the exploitation of their applicability.\</jats:p\>\<jats:p\>This article is categorized under:\<jats:list list-type="simple"\>\<jats:list-item\>\<jats:p\>Data Science \> Artificial Intelligence/Machine Learning\</jats:p\>\</jats:list-item\>\<jats:list-item\>\<jats:p\>Molecular and Statistical Mechanics \> Molecular Interactions\</jats:p\>\</jats:list-item\>\<jats:list-item\>\<jats:p\>Software \> Molecular Modeling\</jats:p\>\</jats:list-item\>\</jats:list\>\</jats:p\>},
keywords = {ANI, Machine learning potential, Review},
pubstate = {published},
tppubtype = {article}
}
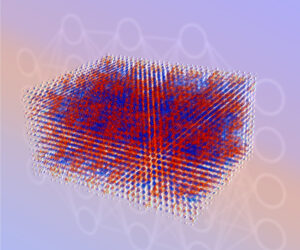
Kulik, H J; Hammerschmidt, T; Schmidt, J; Botti, S; Marques, M A L; Boley, M; Scheffler, M; Todorović, M; Rinke, P; Oses, C; Smolyanyuk, A; Curtarolo, S; Tkatchenko, A; Bartók, A P; Manzhos, S; Ihara, M; Carrington, T; Behler, J; Isayev, O; Veit, M; Grisafi, A; Nigam, J; Ceriotti, M; Schütt, K T; Westermayr, J; Gastegger, M; Maurer, R J; Kalita, B; Burke, K; Nagai, R; Akashi, R; Sugino, O; Hermann, J; Noé, F; Pilati, S; Draxl, C; Kuban, M; Rigamonti, S; Scheidgen, M; Esters, M; Hicks, D; Toher, C; Balachandran, P V; Tamblyn, I; Whitelam, S; Bellinger, C; Ghiringhelli, L M
Roadmap on Machine learning in electronic structure Journal Article
In: Electron. Struct., vol. 4, no. 2, pp. 023004, 2022.
Abstract | Links | BibTeX | Tags: Machine learning potential, Materials informatics, Review
@article{Kulik2022,
title = {Roadmap on Machine learning in electronic structure},
author = {H J Kulik and T Hammerschmidt and J Schmidt and S Botti and M A L Marques and M Boley and M Scheffler and M Todorovi\'{c} and P Rinke and C Oses and A Smolyanyuk and S Curtarolo and A Tkatchenko and A P Bart\'{o}k and S Manzhos and M Ihara and T Carrington and J Behler and O Isayev and M Veit and A Grisafi and J Nigam and M Ceriotti and K T Sch\"{u}tt and J Westermayr and M Gastegger and R J Maurer and B Kalita and K Burke and R Nagai and R Akashi and O Sugino and J Hermann and F No\'{e} and S Pilati and C Draxl and M Kuban and S Rigamonti and M Scheidgen and M Esters and D Hicks and C Toher and P V Balachandran and I Tamblyn and S Whitelam and C Bellinger and L M Ghiringhelli},
doi = {10.1088/2516-1075/ac572f},
year = {2022},
date = {2022-06-01},
urldate = {2022-06-01},
journal = {Electron. Struct.},
volume = {4},
number = {2},
pages = {023004},
publisher = {IOP Publishing},
abstract = {\<jats:title\>Abstract\</jats:title\>\<jats:p\>In recent years, we have been witnessing a paradigm shift in computational materials science. In fact, traditional methods, mostly developed in the second half of the XXth century, are being complemented, extended, and sometimes even completely replaced by faster, simpler, and often more accurate approaches. The new approaches, that we collectively label by machine learning, have their origins in the fields of informatics and artificial intelligence, but are making rapid inroads in all other branches of science. With this in mind, this Roadmap article, consisting of multiple contributions from experts across the field, discusses the use of machine learning in materials science, and share perspectives on current and future challenges in problems as diverse as the prediction of materials properties, the construction of force-fields, the development of exchange correlation functionals for density-functional theory, the solution of the many-body problem, and more. In spite of the already numerous and exciting success stories, we are just at the beginning of a long path that will reshape materials science for the many challenges of the XXIth century.\</jats:p\>},
keywords = {Machine learning potential, Materials informatics, Review},
pubstate = {published},
tppubtype = {article}
}
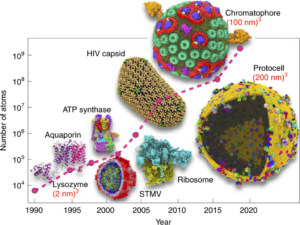
Pandey, Mohit; Fernandez, Michael; Gentile, Francesco; Isayev, Olexandr; Tropsha, Alexander; Stern, Abraham C.; Cherkasov, Artem
The transformational role of GPU computing and deep learning in drug discovery Journal Article
In: Nat Mach Intell, vol. 4, no. 3, pp. 211–221, 2022.
Abstract | Links | BibTeX | Tags: Drug Discovery, Review
@article{Pandey2022,
title = {The transformational role of GPU computing and deep learning in drug discovery},
author = {Mohit Pandey and Michael Fernandez and Francesco Gentile and Olexandr Isayev and Alexander Tropsha and Abraham C. Stern and Artem Cherkasov},
doi = {10.1038/s42256-022-00463-x},
year = {2022},
date = {2022-03-04},
urldate = {2022-03-04},
journal = {Nat Mach Intell},
volume = {4},
number = {3},
pages = {211--221},
publisher = {Springer Science and Business Media LLC},
abstract = {Deep learning has disrupted nearly every field of research, including those of direct importance to drug discovery, such as medicinal chemistry and pharmacology. This revolution has largely been attributed to the unprecedented advances in highly parallelizable graphics processing units (GPUs) and the development of GPU-enabled algorithms. In this Review, we present a comprehensive overview of historical trends and recent advances in GPU algorithms and discuss their immediate impact on the discovery of new drugs and drug targets. We also cover the state-of-the-art of deep learning architectures that have found practical applications in both early drug discovery and consequent hit-to-lead optimization stages, including the acceleration of molecular docking, the evaluation of off-target effects and the prediction of pharmacological properties. We conclude by discussing the impacts of GPU acceleration and deep learning models on the global democratization of the field of drug discovery that may lead to efficient exploration of the ever-expanding chemical universe to accelerate the discovery of novel medicines.},
keywords = {Drug Discovery, Review},
pubstate = {published},
tppubtype = {article}
}
2021
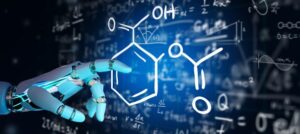
Artrith, Nongnuch; Butler, Keith T.; Coudert, François-Xavier; Han, Seungwu; Isayev, Olexandr; Jain, Anubhav; Walsh, Aron
Best practices in machine learning for chemistry Journal Article
In: Nat. Chem., vol. 13, no. 6, pp. 505–508, 2021, ISSN: 1755-4349.
Abstract | Links | BibTeX | Tags: Machine learning potential, Review
@article{Artrith2021,
title = {Best practices in machine learning for chemistry},
author = {Nongnuch Artrith and Keith T. Butler and Fran\c{c}ois-Xavier Coudert and Seungwu Han and Olexandr Isayev and Anubhav Jain and Aron Walsh},
doi = {10.1038/s41557-021-00716-z},
issn = {1755-4349},
year = {2021},
date = {2021-06-15},
urldate = {2021-06-15},
journal = {Nat. Chem.},
volume = {13},
number = {6},
pages = {505--508},
publisher = {Springer Science and Business Media LLC},
abstract = {Statistical tools based on machine learning are becoming integrated into chemistry research workflows. We discuss the elements necessary to train reliable, repeatable and reproducible models, and recommend a set of guidelines for machine learning reports.},
keywords = {Machine learning potential, Review},
pubstate = {published},
tppubtype = {article}
}