Learning Quantum Mechanics
The high computation cost of quantum mechanical (QM) methods has become a critical bottleneck, limiting a researcher’s abilities to study larger realistic atomistic systems and long-time scales relevant to an experiment. Modern artificial intelligence (AI) also can significantly enhance the role of computers and computational methods in science and engineering. With machines able to learn and offer solutions to some of the most complex physical problems, computational chemistry is well-positioned to be the next frontier for a potential breakthrough. Since 2016, my work has pioneered research at the interface between ML and QM. These studies resulted in developing several families of atomistic molecular ML potentials. All methods were implemented in open-source software and have been made freely available via GitHub. Methods I developed are now used in leading academic laboratories and global companies like Dow Chemical, BASF, GSK, Pfizer, and Genentech.
The success of atomistic ML methods started with a bold idea: can we teach a neural network organic chemistry and quantum mechanics? This led us to develop an “accurate neural network engine for molecular energies” (or ANI for short) potential. To my knowledge, this is the first general-purpose deep neural network for atomistic molecular potential. As of January 2024, the ANI paper was cited over 1500 times. Such a model was trained on a large and diverse dataset of first-principles calculations for small organic molecules. ANI potentials can also utilize information from multiple sources via transfer learning or learning from different types of simulations.
Automating Molecular Discovery
In a recent study, we demonstrated automated experimentation with continuous-flow chemistry fueled by AI can speed up polymer design at an amazing pace. While the automated machinery alone made the experiments faster, the self-learning learning algorithm I developed made the experiments drastically more efficient by narrowing down the field of potential candidates as the experiment progressed. We showed that a properly trained AI model can mimic expert chemists’ skills. This is a prime example of the transfer of the decision power from human experts to AI. Given the expertise of my laboratory and unique positioning, I am taking on a challenge to revolutionize how chemical research and education are done, accelerating the pace of discovery as demanded by the global challenges our society is facing.
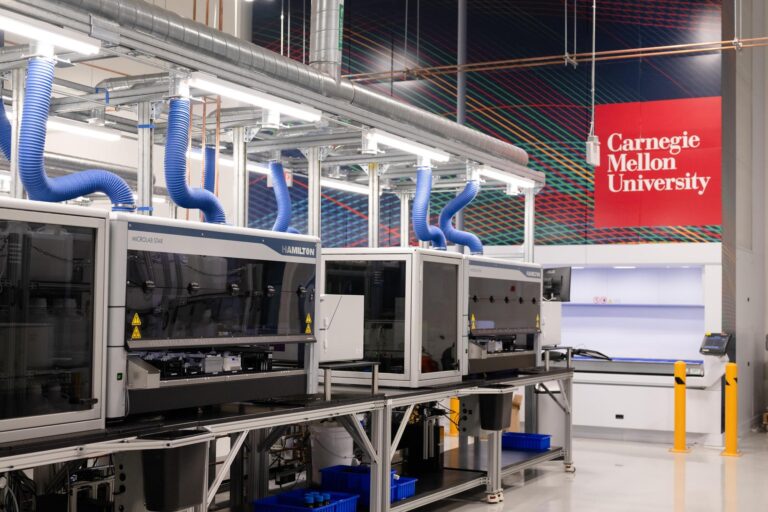
Within the next few years, I would like to push the frontiers of computational chemistry methods and AI to mimic and then supersede the chemical intuition and decision-making of expert scientists. The critical need for “Chemical Intelligence” exemplifies the need for advancement beyond the presently available algorithms in two primary ways:
- elevate machine learning from generating data models toward generating expert-approved inferences and conclusions;
- enable autonomous reasoning about these outcomes and prior data to generate and execute an actionable research plan.