2024
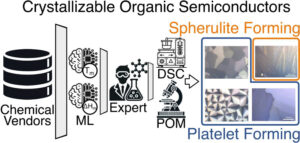
Johnson, Holly M.; Gusev, Filipp; Dull, Jordan T.; Seo, Yejoon; Priestley, Rodney D.; Isayev, Olexandr; Rand, Barry P.
Discovery of Crystallizable Organic Semiconductors with Machine Learning Journal Article
In: J. Am. Chem. Soc., vol. 146, no. 31, pp. 21583–21590, 2024, ISSN: 1520-5126.
Abstract | Links | BibTeX | Tags: Active learning, Crystal structure
@article{Johnson2024,
title = {Discovery of Crystallizable Organic Semiconductors with Machine Learning},
author = {Holly M. Johnson and Filipp Gusev and Jordan T. Dull and Yejoon Seo and Rodney D. Priestley and Olexandr Isayev and Barry P. Rand},
url = {https://olexandrisayev.com/wp-content/uploads/johnson-et-al-2024-discovery-of-crystallizable-organic-semiconductors-with-machine-learning-1.pdf},
doi = {10.1021/jacs.4c05245},
issn = {1520-5126},
year = {2024},
date = {2024-08-07},
urldate = {2024-08-07},
journal = {J. Am. Chem. Soc.},
volume = {146},
number = {31},
pages = {21583--21590},
publisher = {American Chemical Society (ACS)},
abstract = {Crystalline organic semiconductors are known to have improved charge carrier mobility and exciton diffusion length in comparison to their amorphous counterparts. Certain organic molecular thin films can be transitioned from initially prepared amorphous layers to large-scale crystalline films via abrupt thermal annealing. Ideally, these films crystallize as platelets with long-range-ordered domains on the scale of tens to hundreds of microns. However, other organic molecular thin films may instead crystallize as spherulites or resist crystallization entirely. Organic molecules that have the capability of transforming into a platelet morphology feature both high melting point (Tm) and crystallization driving force (ΔGc). In this work, we employed machine learning (ML) to identify candidate organic materials with the potential to crystallize into platelets by estimating the aforementioned thermal properties. Six organic molecules identified by the ML algorithm were experimentally evaluated; three crystallized as platelets, one crystallized as a spherulite, and two resisted thin film crystallization. These results demonstrate a successful application of ML in the scope of predicting thermal properties of organic molecules and reinforce the principles of Tm and ΔGc as metrics that aid in predicting the crystallization behavior of organic thin films.},
keywords = {Active learning, Crystal structure},
pubstate = {published},
tppubtype = {article}
}
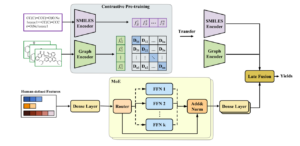
Chen, Jiayuan; Guo, Kehan; Liu, Zhen; Isayev, Olexandr; Zhang, Xiangliang
Uncertainty-Aware Yield Prediction with Multimodal Molecular Features Journal Article
In: AAAI, vol. 38, no. 8, pp. 8274–8282, 2024, ISSN: 2374-3468.
Abstract | Links | BibTeX | Tags: Organic reactions
@article{Chen2024,
title = {Uncertainty-Aware Yield Prediction with Multimodal Molecular Features},
author = {Jiayuan Chen and Kehan Guo and Zhen Liu and Olexandr Isayev and Xiangliang Zhang},
doi = {10.1609/aaai.v38i8.28668},
issn = {2374-3468},
year = {2024},
date = {2024-03-25},
urldate = {2024-03-25},
journal = {AAAI},
volume = {38},
number = {8},
pages = {8274--8282},
publisher = {Association for the Advancement of Artificial Intelligence (AAAI)},
abstract = {\<jats:p\>Predicting chemical reaction yields is pivotal for efficient chemical synthesis, an area that focuses on the creation of novel compounds for diverse uses.
Yield prediction demands accurate representations of reactions for forecasting practical transformation rates. Yet, the uncertainty issues broadcasting in real-world situations prohibit current models to excel in this task owing to the high sensitivity of yield activities and the uncertainty in yield measurements. Existing models often utilize single-modal feature representations, such as molecular fingerprints, SMILES sequences, or molecular graphs, which is not sufficient to capture the complex interactions and dynamic behavior of molecules in reactions. In this paper, we present an advanced Uncertainty-Aware Multimodal model (UAM) to tackle these challenges. Our approach seamlessly integrates data sources from multiple modalities by encompassing sequence representations, molecular graphs, and expert-defined chemical reaction features for a comprehensive representation of reactions. Additionally, we address both the model and data-based uncertainty, refining the model\'s predictive capability. Extensive experiments on three datasets, including two high throughput experiment (HTE) datasets and one chemist-constructed Amide coupling reaction dataset, demonstrate that UAM outperforms the state-of-the-art methods. The code and used datasets are available at https://github.com/jychen229/Multimodal-reaction-yield-prediction.\</jats:p\>},
keywords = {Organic reactions},
pubstate = {published},
tppubtype = {article}
}
Yield prediction demands accurate representations of reactions for forecasting practical transformation rates. Yet, the uncertainty issues broadcasting in real-world situations prohibit current models to excel in this task owing to the high sensitivity of yield activities and the uncertainty in yield measurements. Existing models often utilize single-modal feature representations, such as molecular fingerprints, SMILES sequences, or molecular graphs, which is not sufficient to capture the complex interactions and dynamic behavior of molecules in reactions. In this paper, we present an advanced Uncertainty-Aware Multimodal model (UAM) to tackle these challenges. Our approach seamlessly integrates data sources from multiple modalities by encompassing sequence representations, molecular graphs, and expert-defined chemical reaction features for a comprehensive representation of reactions. Additionally, we address both the model and data-based uncertainty, refining the model's predictive capability. Extensive experiments on three datasets, including two high throughput experiment (HTE) datasets and one chemist-constructed Amide coupling reaction dataset, demonstrate that UAM outperforms the state-of-the-art methods. The code and used datasets are available at https://github.com/jychen229/Multimodal-reaction-yield-prediction.</jats:p>
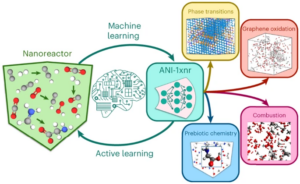
Zhang, Shuhao; Makoś, Małgorzata Z.; Jadrich, Ryan B.; Kraka, Elfi; Barros, Kipton; Nebgen, Benjamin T.; Tretiak, Sergei; Isayev, Olexandr; Lubbers, Nicholas; Messerly, Richard A.; Smith, Justin S.
Exploring the frontiers of condensed-phase chemistry with a general reactive machine learning potential Journal Article
In: Nat. Chem., 2024.
Abstract | Links | BibTeX | Tags: Active learning, ANI, Organic reactions
@article{Zhang2024,
title = {Exploring the frontiers of condensed-phase chemistry with a general reactive machine learning potential},
author = {Shuhao Zhang and Ma\lgorzata Z. Mako\'{s} and Ryan B. Jadrich and Elfi Kraka and Kipton Barros and Benjamin T. Nebgen and Sergei Tretiak and Olexandr Isayev and Nicholas Lubbers and Richard A. Messerly and Justin S. Smith},
doi = {10.1038/s41557-023-01427-3},
year = {2024},
date = {2024-03-07},
urldate = {2024-03-07},
journal = {Nat. Chem.},
publisher = {Springer Science and Business Media LLC},
abstract = {Atomistic simulation has a broad range of applications from drug design to materials discovery. Machine learning interatomic potentials (MLIPs) have become an efficient alternative to computationally expensive ab initio simulations. For this reason, chemistry and materials science would greatly benefit from a general reactive MLIP, that is, an MLIP that is applicable to a broad range of reactive chemistry without the need for refitting. Here we develop a general reactive MLIP (ANI-1xnr) through automated sampling of condensed-phase reactions. ANI-1xnr is then applied to study five distinct systems: carbon solid-phase nucleation, graphene ring formation from acetylene, biofuel additives, combustion of methane and the spontaneous formation of glycine from early earth small molecules. In all studies, ANI-1xnr closely matches experiment (when available) and/or previous studies using traditional model chemistry methods. As such, ANI-1xnr proves to be a highly general reactive MLIP for C, H, N and O elements in the condensed phase, enabling high-throughput in silico reactive chemistry experimentation.},
keywords = {Active learning, ANI, Organic reactions},
pubstate = {published},
tppubtype = {article}
}
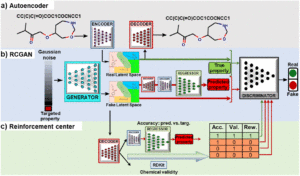
Sattari, Kianoosh; Li, Dawei; Kalita, Bhupalee; Xie, Yunchao; Lighvan, Fatemeh Barmaleki; Isayev, Olexandr; Lin, Jian
De novo molecule design towards biased properties via a deep generative framework and iterative transfer learning Journal Article
In: Digital Discovery, vol. 3, no. 2, pp. 410–421, 2024.
Abstract | Links | BibTeX | Tags: Active learning, Generative AI
@article{Sattari2024,
title = {\textit{De novo} molecule design towards biased properties \textit{via} a deep generative framework and iterative transfer learning},
author = {Kianoosh Sattari and Dawei Li and Bhupalee Kalita and Yunchao Xie and Fatemeh Barmaleki Lighvan and Olexandr Isayev and Jian Lin},
doi = {10.1039/d3dd00210a},
year = {2024},
date = {2024-02-14},
urldate = {2024-02-14},
journal = {Digital Discovery},
volume = {3},
number = {2},
pages = {410--421},
publisher = {Royal Society of Chemistry (RSC)},
abstract = {De novo design of molecules with targeted properties represents a new frontier in molecule development. Despite enormous progress, two main challenges remain: (i) generating novel molecules conditioned on targeted, continuous property values; (ii) obtaining molecules with property values beyond the range in the training data. To tackle these challenges, we propose a reinforced regressional and conditional generative adversarial network (RRCGAN) to generate chemically valid molecules with targeted HOMO\textendashLUMO energy gap (ΔEH\textendashL) as a proof-of-concept study. As validated by density functional theory (DFT) calculation, 75% of the generated molecules have a relative error (RE) of \<20% of the targeted ΔEH\textendashL values. To bias the generation toward the ΔEH\textendashL values beyond the range of the original training molecules, transfer learning was applied to iteratively retrain the RRCGAN model. After just two iterations, the mean ΔEH\textendashL of the generated molecules increases to 8.7 eV from the mean value of 5.9 eV shown in the initial training dataset. Qualitative and quantitative analyses reveal that the model has successfully captured the underlying structure\textendashproperty relationship, which agrees well with the established physical and chemical rules. These results present a trustworthy, purely data-driven methodology for the highly efficient generation of novel molecules with different targeted properties.},
keywords = {Active learning, Generative AI},
pubstate = {published},
tppubtype = {article}
}
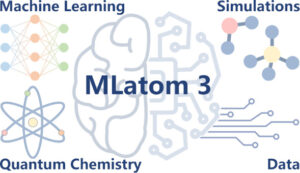
Dral, Pavlo O.; Ge, Fuchun; Hou, Yi-Fan; Zheng, Peikun; Chen, Yuxinxin; Barbatti, Mario; Isayev, Olexandr; Wang, Cheng; Xue, Bao-Xin; Jr, Max Pinheiro; Su, Yuming; Dai, Yiheng; Chen, Yangtao; Zhang, Lina; Zhang, Shuang; Ullah, Arif; Zhang, Quanhao; Ou, Yanchi
MLatom 3: A Platform for Machine Learning-Enhanced Computational Chemistry Simulations and Workflows Journal Article
In: J. Chem. Theory Comput., vol. 20, no. 3, pp. 1193–1213, 2024.
Abstract | Links | BibTeX | Tags: ANI, Machine learning potential
@article{Dral2024,
title = {MLatom 3: A Platform for Machine Learning-Enhanced Computational Chemistry Simulations and Workflows},
author = {Pavlo O. Dral and Fuchun Ge and Yi-Fan Hou and Peikun Zheng and Yuxinxin Chen and Mario Barbatti and Olexandr Isayev and Cheng Wang and Bao-Xin Xue and Max Pinheiro Jr and Yuming Su and Yiheng Dai and Yangtao Chen and Lina Zhang and Shuang Zhang and Arif Ullah and Quanhao Zhang and Yanchi Ou},
doi = {10.1021/acs.jctc.3c01203},
year = {2024},
date = {2024-02-13},
urldate = {2024-02-13},
journal = {J. Chem. Theory Comput.},
volume = {20},
number = {3},
pages = {1193--1213},
publisher = {American Chemical Society (ACS)},
abstract = {Machine learning (ML) is increasingly becoming a common tool in computational chemistry. At the same time, the rapid development of ML methods requires a flexible software framework for designing custom workflows. MLatom 3 is a program package designed to leverage the power of ML to enhance typical computational chemistry simulations and to create complex workflows. This open-source package provides plenty of choice to the users who can run simulations with the command-line options, input files, or with scripts using MLatom as a Python package, both on their computers and on the online XACS cloud computing service at XACScloud.com. Computational chemists can calculate energies and thermochemical properties, optimize geometries, run molecular and quantum dynamics, and simulate (ro)vibrational, one-photon UV/vis absorption, and two-photon absorption spectra with ML, quantum mechanical, and combined models. The users can choose from an extensive library of methods containing pretrained ML models and quantum mechanical approximations such as AIQM1 approaching coupled-cluster accuracy. The developers can build their own models using various ML algorithms. The great flexibility of MLatom is largely due to the extensive use of the interfaces to many state-of-the-art software packages and libraries.},
keywords = {ANI, Machine learning potential},
pubstate = {published},
tppubtype = {article}
}
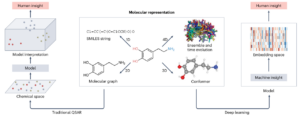
Tropsha, Alexander; Isayev, Olexandr; Varnek, Alexandre; Schneider, Gisbert; Cherkasov, Artem
Integrating QSAR modelling and deep learning in drug discovery: the emergence of deep QSAR Journal Article
In: Nat Rev Drug Discov, vol. 23, no. 2, pp. 141–155, 2024.
Abstract | Links | BibTeX | Tags: Drug Discovery, Generative AI, Review
@article{Tropsha2023,
title = {Integrating QSAR modelling and deep learning in drug discovery: the emergence of deep QSAR},
author = {Alexander Tropsha and Olexandr Isayev and Alexandre Varnek and Gisbert Schneider and Artem Cherkasov},
doi = {10.1038/s41573-023-00832-0},
year = {2024},
date = {2024-01-12},
urldate = {2024-01-12},
journal = {Nat Rev Drug Discov},
volume = {23},
number = {2},
pages = {141--155},
publisher = {Springer Science and Business Media LLC},
abstract = {Quantitative structure\textendashactivity relationship (QSAR) modelling, an approach that was introduced 60 years ago, is widely used in computer-aided drug design. In recent years, progress in artificial intelligence techniques, such as deep learning, the rapid growth of databases of molecules for virtual screening and dramatic improvements in computational power have supported the emergence of a new field of QSAR applications that we term ‘deep QSAR’. Marking a decade from the pioneering applications of deep QSAR to tasks involved in small-molecule drug discovery, we herein describe key advances in the field, including deep generative and reinforcement learning approaches in molecular design, deep learning models for synthetic planning and the application of deep QSAR models in structure-based virtual screening. We also reflect on the emergence of quantum computing, which promises to further accelerate deep QSAR applications and the need for open-source and democratized resources to support computer-aided drug design.},
keywords = {Drug Discovery, Generative AI, Review},
pubstate = {published},
tppubtype = {article}
}
2023
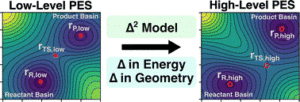
Zhao, Qiyuan; Anstine, Dylan M.; Isayev, Olexandr; Savoie, Brett M.
Δ2 machine learning for reaction property prediction Journal Article
In: Chem. Sci., vol. 14, no. 46, pp. 13392–13401, 2023.
Abstract | Links | BibTeX | Tags: AIMNet, Machine learning potential, Organic reactions
@article{Zhao2023b,
title = {Δ^{2} machine learning for reaction property prediction},
author = {Qiyuan Zhao and Dylan M. Anstine and Olexandr Isayev and Brett M. Savoie},
doi = {10.1039/d3sc02408c},
year = {2023},
date = {2023-11-29},
urldate = {2023-11-29},
journal = {Chem. Sci.},
volume = {14},
number = {46},
pages = {13392--13401},
publisher = {Royal Society of Chemistry (RSC)},
abstract = {The emergence of Δ-learning models, whereby machine learning (ML) is used to predict a correction to a low-level energy calculation, provides a versatile route to accelerate high-level energy evaluations at a given geometry. However, Δ-learning models are inapplicable to reaction properties like heats of reaction and activation energies that require both a high-level geometry and energy evaluation. Here, a Δ2-learning model is introduced that can predict high-level activation energies based on low-level critical-point geometries. The Δ2 model uses an atom-wise featurization typical of contemporary ML interatomic potentials (MLIPs) and is trained on a dataset of ∼167 000 reactions, using the GFN2-xTB energy and critical-point geometry as a low-level input and the B3LYP-D3/TZVP energy calculated at the B3LYP-D3/TZVP critical point as a high-level target. The excellent performance of the Δ2 model on unseen reactions demonstrates the surprising ease with which the model implicitly learns the geometric deviations between the low-level and high-level geometries that condition the activation energy prediction. The transferability of the Δ2 model is validated on several external testing sets where it shows near chemical accuracy, illustrating the benefits of combining ML models with readily available physical-based information from semi-empirical quantum chemistry calculations. Fine-tuning of the Δ2 model on a small number of Gaussian-4 calculations produced a 35% accuracy improvement over DFT activation energy predictions while retaining xTB-level cost. The Δ2 model approach proves to be an efficient strategy for accelerating chemical reaction characterization with minimal sacrifice in prediction accuracy.},
keywords = {AIMNet, Machine learning potential, Organic reactions},
pubstate = {published},
tppubtype = {article}
}
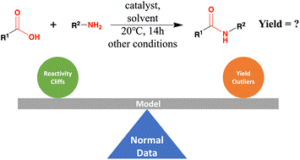
Liu, Zhen; Moroz, Yurii S.; Isayev, Olexandr
The challenge of balancing model sensitivity and robustness in predicting yields: a benchmarking study of amide coupling reactions Journal Article
In: Chem. Sci., vol. 14, no. 39, pp. 10835–10846, 2023.
Abstract | Links | BibTeX | Tags: AIMNet, Organic reactions
@article{Liu2023,
title = {The challenge of balancing model sensitivity and robustness in predicting yields: a benchmarking study of amide coupling reactions},
author = {Zhen Liu and Yurii S. Moroz and Olexandr Isayev},
doi = {10.1039/d3sc03902a},
year = {2023},
date = {2023-10-11},
urldate = {2023-10-11},
journal = {Chem. Sci.},
volume = {14},
number = {39},
pages = {10835--10846},
publisher = {Royal Society of Chemistry (RSC)},
abstract = {Accurate prediction of reaction yield is the holy grail for computer-assisted synthesis prediction, but current models have failed to generalize to large literature datasets. To understand the causes and inspire future design, we systematically benchmarked the yield prediction task. We carefully curated and augmented a literature dataset of 41 239 amide coupling reactions, each with information on reactants, products, intermediates, yields, and reaction contexts, and provided 3D structures for the molecules. We calculated molecular features related to 2D and 3D structure information, as well as physical and electronic properties. These descriptors were paired with 4 categories of machine learning methods (linear, kernel, ensemble, and neural network), yielding valuable benchmarks about feature and model performance. Despite the excellent performance on a high-throughput experiment (HTE) dataset (R2 around 0.9), no method gave satisfactory results on the literature data. The best performance was an R2 of 0.395 ± 0.020 using the stack technique. Error analysis revealed that reactivity cliff and yield uncertainty are among the main reasons for incorrect predictions. Removing reactivity cliffs and uncertain reactions boosted the R2 to 0.457 ± 0.006. These results highlight that yield prediction models must be sensitive to the reactivity change due to the subtle structure variance, as well as be robust to the uncertainty associated with yield measurements.},
keywords = {AIMNet, Organic reactions},
pubstate = {published},
tppubtype = {article}
}
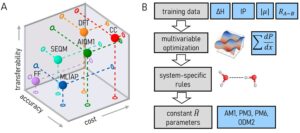
Fedik, Nikita; Nebgen, Benjamin; Lubbers, Nicholas; Barros, Kipton; Kulichenko, Maksim; Li, Ying Wai; Zubatyuk, Roman; Messerly, Richard; Isayev, Olexandr; Tretiak, Sergei
Synergy of semiempirical models and machine learning in computational chemistry Journal Article
In: J. Chem. Phys., vol. 159, no. 11, pp. 110901 , 2023.
Abstract | Links | BibTeX | Tags: Machine learning potential, Review
@article{Fedik2023,
title = {Synergy of semiempirical models and machine learning in computational chemistry},
author = {Nikita Fedik and Benjamin Nebgen and Nicholas Lubbers and Kipton Barros and Maksim Kulichenko and Ying Wai Li and Roman Zubatyuk and Richard Messerly and Olexandr Isayev and Sergei Tretiak},
doi = {10.1063/5.0151833},
year = {2023},
date = {2023-09-21},
urldate = {2023-09-21},
journal = {J. Chem. Phys.},
volume = {159},
number = {11},
pages = {110901 },
publisher = {AIP Publishing},
abstract = {Catalyzed by enormous success in the industrial sector, many research programs have been exploring data-driven, machine learning approaches. Performance can be poor when the model is extrapolated to new regions of chemical space, e.g., new bonding types, new many-body interactions. Another important limitation is the spatial locality assumption in model architecture, and this limitation cannot be overcome with larger or more diverse datasets. The outlined challenges are primarily associated with the lack of electronic structure information in surrogate models such as interatomic potentials. Given the fast development of machine learning and computational chemistry methods, we expect some limitations of surrogate models to be addressed in the near future; nevertheless spatial locality assumption will likely remain a limiting factor for their transferability. Here, we suggest focusing on an equally important effort\textemdashdesign of physics-informed models that leverage the domain knowledge and employ machine learning only as a corrective tool. In the context of material science, we will focus on semi-empirical quantum mechanics, using machine learning to predict corrections to the reduced-order Hamiltonian model parameters. The resulting models are broadly applicable, retain the speed of semiempirical chemistry, and frequently achieve accuracy on par with much more expensive ab initio calculations. These early results indicate that future work, in which machine learning and quantum chemistry methods are developed jointly, may provide the best of all worlds for chemistry applications that demand both high accuracy and high numerical efficiency.},
keywords = {Machine learning potential, Review},
pubstate = {published},
tppubtype = {article}
}
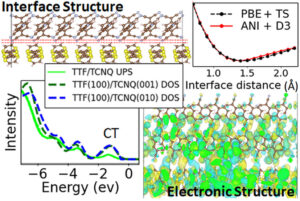
Moayedpour, Saeed; Bier, Imanuel; Wen, Wen; Dardzinski, Derek; Isayev, Olexandr; Marom, Noa
Structure Prediction of Epitaxial Organic Interfaces with Ogre, Demonstrated for Tetracyanoquinodimethane (TCNQ) on Tetrathiafulvalene (TTF) Journal Article
In: J. Phys. Chem. C, vol. 127, no. 21, pp. 10398–10410, 2023.
Abstract | Links | BibTeX | Tags: Crystal structure, Machine learning potential
@article{Moayedpour2023,
title = {Structure Prediction of Epitaxial Organic Interfaces with Ogre, Demonstrated for Tetracyanoquinodimethane (TCNQ) on Tetrathiafulvalene (TTF)},
author = {Saeed Moayedpour and Imanuel Bier and Wen Wen and Derek Dardzinski and Olexandr Isayev and Noa Marom},
doi = {10.1021/acs.jpcc.3c02384},
year = {2023},
date = {2023-06-01},
urldate = {2023-06-01},
journal = {J. Phys. Chem. C},
volume = {127},
number = {21},
pages = {10398--10410},
publisher = {American Chemical Society (ACS)},
abstract = {Highly ordered epitaxial interfaces between organic semiconductors are considered as a promising avenue for enhancing the performance of organic electronic devices including solar cells and transistors, thanks to their well-controlled, uniform electronic properties and high carrier mobilities. The electronic structure of epitaxial organic interfaces and their functionality in devices are inextricably linked to their structure. We present a method for structure prediction of epitaxial organic interfaces based on lattice matching followed by surface matching, implemented in the open-source Python package, Ogre. The lattice matching step produces domain-matched interfaces, where commensurability is achieved with different integer multiples of the substrate and film unit cells. In the surface matching step, Bayesian optimization (BO) is used to find the interfacial distance and registry between the substrate and film. The BO objective function is based on dispersion corrected deep neural network interatomic potentials. These are shown to be in qualitative agreement with density functional theory (DFT) regarding the optimal position of the film on top of the substrate and the ranking of putative interface structures. Ogre is used to investigate the epitaxial interface of 7,7,8,8-tetracyanoquinodimethane (TCNQ) on tetrathiafulvalene (TTF), whose electronic structure has been probed by ultraviolet photoemission spectroscopy (UPS), but whose structure had been hitherto unknown [Organic Electronics 2017, 48, 371]. We find that TCNQ(001) on top of TTF(100) is the most stable interface configuration, closely followed by TCNQ(010) on top of TTF(100). The density of states, calculated using DFT, is in excellent agreement with UPS, including the presence of an interface charge transfer state.},
keywords = {Crystal structure, Machine learning potential},
pubstate = {published},
tppubtype = {article}
}
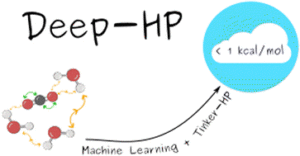
Inizan, Théo Jaffrelot; Plé, Thomas; Adjoua, Olivier; Ren, Pengyu; Gökcan, Hatice; Isayev, Olexandr; Lagardère, Louis; Piquemal, Jean-Philip
Scalable hybrid deep neural networks/polarizable potentials biomolecular simulations including long-range effects Journal Article
In: Chem. Sci., vol. 14, no. 20, pp. 5438–5452, 2023.
Abstract | Links | BibTeX | Tags: ANI, Machine learning potential
@article{JaffrelotInizan2023,
title = {Scalable hybrid deep neural networks/polarizable potentials biomolecular simulations including long-range effects},
author = {Th\'{e}o Jaffrelot Inizan and Thomas Pl\'{e} and Olivier Adjoua and Pengyu Ren and Hatice G\"{o}kcan and Olexandr Isayev and Louis Lagard\`{e}re and Jean-Philip Piquemal},
doi = {10.1039/d2sc04815a},
year = {2023},
date = {2023-05-24},
urldate = {2023-05-24},
journal = {Chem. Sci.},
volume = {14},
number = {20},
pages = {5438--5452},
publisher = {Royal Society of Chemistry (RSC)},
abstract = {\<jats:p\>Deep-HP is a scalable extension of the Tinker-HP multi-GPU molecular dynamics (MD) package enabling the use of Pytorch/TensorFlow Deep Neural Network (DNN) models.\</jats:p\>},
keywords = {ANI, Machine learning potential},
pubstate = {published},
tppubtype = {article}
}
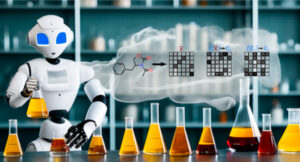
Anstine, Dylan M.; Isayev, Olexandr
Generative Models as an Emerging Paradigm in the Chemical Sciences Journal Article
In: J. Am. Chem. Soc., vol. 145, no. 16, pp. 8736–8750, 2023.
Abstract | Links | BibTeX | Tags: Drug Discovery, Generative AI, Review, RL
@article{Anstine2023b,
title = {Generative Models as an Emerging Paradigm in the Chemical Sciences},
author = {Dylan M. Anstine and Olexandr Isayev},
doi = {10.1021/jacs.2c13467},
year = {2023},
date = {2023-04-26},
urldate = {2023-04-26},
journal = {J. Am. Chem. Soc.},
volume = {145},
number = {16},
pages = {8736--8750},
publisher = {American Chemical Society (ACS)},
abstract = {Traditional computational approaches to design chemical species are limited by the need to compute properties for a vast number of candidates, e.g., by discriminative modeling. Therefore, inverse design methods aim to start from the desired property and optimize a corresponding chemical structure. From a machine learning viewpoint, the inverse design problem can be addressed through so-called generative modeling. Mathematically, discriminative models are defined by learning the probability distribution function of properties given the molecular or material structure. In contrast, a generative model seeks to exploit the joint probability of a chemical species with target characteristics. The overarching idea of generative modeling is to implement a system that produces novel compounds that are expected to have a desired set of chemical features, effectively sidestepping issues found in the forward design process. In this contribution, we overview and critically analyze popular generative algorithms like generative adversarial networks, variational autoencoders, flow, and diffusion models. We highlight key differences between each of the models, provide insights into recent success stories, and discuss outstanding challenges for realizing generative modeling discovered solutions in chemical applications.},
keywords = {Drug Discovery, Generative AI, Review, RL},
pubstate = {published},
tppubtype = {article}
}
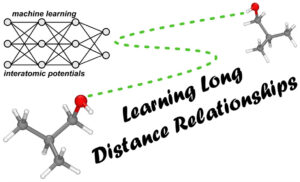
Anstine, Dylan M.; Isayev, Olexandr
Machine Learning Interatomic Potentials and Long-Range Physics Journal Article
In: J. Phys. Chem. A, vol. 127, no. 11, pp. 2417–2431, 2023, ISSN: 1520-5215.
Abstract | Links | BibTeX | Tags: AIMNet, ANI, Machine learning potential, Review
@article{Anstine2023,
title = {Machine Learning Interatomic Potentials and Long-Range Physics},
author = {Dylan M. Anstine and Olexandr Isayev},
doi = {10.1021/acs.jpca.2c06778},
issn = {1520-5215},
year = {2023},
date = {2023-03-23},
urldate = {2023-03-23},
journal = {J. Phys. Chem. A},
volume = {127},
number = {11},
pages = {2417--2431},
publisher = {American Chemical Society (ACS)},
abstract = {Advances in machine learned interatomic potentials (MLIPs), such as those using neural networks, have resulted in short-range models that can infer interaction energies with near ab initio accuracy and orders of magnitude reduced computational cost. For many atom systems, including macromolecules, biomolecules, and condensed matter, model accuracy can become reliant on the description of short- and long-range physical interactions. The latter terms can be difficult to incorporate into an MLIP framework. Recent research has produced numerous models with considerations for nonlocal electrostatic and dispersion interactions, leading to a large range of applications that can be addressed using MLIPs. In light of this, we present a Perspective focused on key methodologies and models being used where the presence of nonlocal physics and chemistry are crucial for describing system properties. The strategies covered include MLIPs augmented with dispersion corrections, electrostatics calculated with charges predicted from atomic environment descriptors, the use of self-consistency and message passing iterations to propagated nonlocal system information, and charges obtained via equilibration schemes. We aim to provide a pointed discussion to support the development of machine learning-based interatomic potentials for systems where contributions from only nearsighted terms are deficient.},
keywords = {AIMNet, ANI, Machine learning potential, Review},
pubstate = {published},
tppubtype = {article}
}
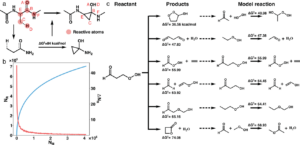
Zhao, Qiyuan; Vaddadi, Sai Mahit; Woulfe, Michael; Ogunfowora, Lawal A.; Garimella, Sanjay S.; Isayev, Olexandr; Savoie, Brett M.
Comprehensive exploration of graphically defined reaction spaces Journal Article
In: Sci Data, vol. 10, pp. 145 , 2023.
Abstract | Links | BibTeX | Tags: dataset, Organic reactions
@article{Zhao2023,
title = {Comprehensive exploration of graphically defined reaction spaces},
author = {Qiyuan Zhao and Sai Mahit Vaddadi and Michael Woulfe and Lawal A. Ogunfowora and Sanjay S. Garimella and Olexandr Isayev and Brett M. Savoie},
doi = {10.1038/s41597-023-02043-z},
year = {2023},
date = {2023-03-15},
urldate = {2023-03-15},
journal = {Sci Data},
volume = {10},
pages = {145 },
publisher = {Springer Science and Business Media LLC},
abstract = {Existing reaction transition state (TS) databases are comparatively small and lack chemical diversity. Here, this data gap has been addressed using the concept of a graphically-defined model reaction to comprehensively characterize a reaction space associated with C, H, O, and N containing molecules with up to 10 heavy (non-hydrogen) atoms. The resulting dataset is composed of 176,992 organic reactions possessing at least one validated TS, activation energy, heat of reaction, reactant and product geometries, frequencies, and atom-mapping. For 33,032 reactions, more than one TS was discovered by conformational sampling, allowing conformational errors in TS prediction to be assessed. Data is supplied at the GFN2-xTB and B3LYP-D3/TZVP levels of theory. A subset of reactions were recalculated at the CCSD(T)-F12/cc-pVDZ-F12 and ωB97X-D2/def2-TZVP levels to establish relative errors. The resulting collection of reactions and properties are called the Reaction Graph Depth 1 (RGD1) dataset. RGD1 represents the largest and most chemically diverse TS dataset published to date and should find immediate use in developing novel machine learning models for predicting reaction properties.},
keywords = {dataset, Organic reactions},
pubstate = {published},
tppubtype = {article}
}
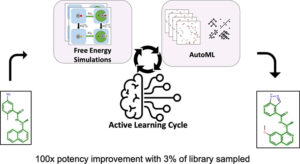
Gusev, Filipp; Gutkin, Evgeny; Kurnikova, Maria G.; Isayev, Olexandr
Active Learning Guided Drug Design Lead Optimization Based on Relative Binding Free Energy Modeling Journal Article
In: J. Chem. Inf. Model., vol. 63, no. 2, pp. 583–594, 2023.
Abstract | Links | BibTeX | Tags: Active learning, Drug Discovery
@article{Gusev2023,
title = {Active Learning Guided Drug Design Lead Optimization Based on Relative Binding Free Energy Modeling},
author = {Filipp Gusev and Evgeny Gutkin and Maria G. Kurnikova and Olexandr Isayev},
doi = {10.1021/acs.jcim.2c01052},
year = {2023},
date = {2023-01-23},
urldate = {2023-01-23},
journal = {J. Chem. Inf. Model.},
volume = {63},
number = {2},
pages = {583--594},
publisher = {American Chemical Society (ACS)},
abstract = {In silico identification of potent protein inhibitors commonly requires prediction of a ligand binding free energy (BFE). Thermodynamics integration (TI) based on molecular dynamics (MD) simulations is a BFE calculation method capable of acquiring accurate BFE, but it is computationally expensive and time-consuming. In this work, we have developed an efficient automated workflow for identifying compounds with the lowest BFE among thousands of congeneric ligands, which requires only hundreds of TI calculations. Automated machine learning (AutoML) orchestrated by active learning (AL) in an AL\textendashAutoML workflow allows unbiased and efficient search for a small set of best-performing molecules. We have applied this workflow to select inhibitors of the SARS-CoV-2 papain-like protease and were able to find 133 compounds with improved binding affinity, including 16 compounds with better than 100-fold binding affinity improvement. We obtained a hit rate that outperforms that expected of traditional expert medicinal chemist-guided campaigns. Thus, we demonstrate that the combination of AL and AutoML with free energy simulations provides at least 20× speedup relative to the na\"{i}ve brute force approaches.},
keywords = {Active learning, Drug Discovery},
pubstate = {published},
tppubtype = {article}
}
2022
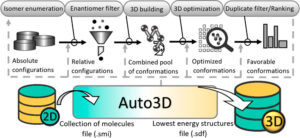
Liu, Zhen; Zubatiuk, Tetiana; Roitberg, Adrian; Isayev, Olexandr
Auto3D: Automatic Generation of the Low-Energy 3D Structures with ANI Neural Network Potentials Journal Article
In: J. Chem. Inf. Model., vol. 62, no. 22, pp. 5373–5382, 2022.
Abstract | Links | BibTeX | Tags: ANI, Drug Discovery
@article{Liu2022,
title = {Auto3D: Automatic Generation of the Low-Energy 3D Structures with ANI Neural Network Potentials},
author = {Zhen Liu and Tetiana Zubatiuk and Adrian Roitberg and Olexandr Isayev},
doi = {10.1021/acs.jcim.2c00817},
year = {2022},
date = {2022-11-28},
urldate = {2022-11-28},
journal = {J. Chem. Inf. Model.},
volume = {62},
number = {22},
pages = {5373--5382},
publisher = {American Chemical Society (ACS)},
abstract = {Computational programs accelerate the chemical discovery processes but often need proper three-dimensional molecular information as part of the input. Getting optimal molecular structures is challenging because it requires enumerating and optimizing a huge space of stereoisomers and conformers. We developed the Python-based Auto3D package for generating the low-energy 3D structures using SMILES as the input. Auto3D is based on state-of-the-art algorithms and can automatize the isomer enumeration and duplicate filtering process, 3D building process, geometry optimization, and ranking process. Tested on 50 molecules with multiple unspecified stereocenters, Auto3D is guaranteed to find the stereoconfiguration that yields the lowest-energy conformer. With Auto3D, we provide an extension of the ANI model. The new model, dubbed ANI-2xt, is trained on a tautomer-rich data set. ANI-2xt is benchmarked with DFT methods on geometry optimization and electronic and Gibbs free energy calculations. Compared with ANI-2x, ANI-2xt provides a 42% error reduction for tautomeric reaction energy calculations when using the gold-standard coupled-cluster calculation as the reference. ANI-2xt can accurately predict the energies and is several orders of magnitude faster than DFT methods.},
keywords = {ANI, Drug Discovery},
pubstate = {published},
tppubtype = {article}
}
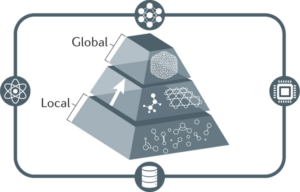
Fedik, Nikita; Zubatyuk, Roman; Kulichenko, Maksim; Lubbers, Nicholas; Smith, Justin S.; Nebgen, Benjamin; Messerly, Richard; Li, Ying Wai; Boldyrev, Alexander I.; Barros, Kipton; Isayev, Olexandr; Tretiak, Sergei
Extending machine learning beyond interatomic potentials for predicting molecular properties Journal Article
In: Nat Rev Chem, vol. 6, no. 9, pp. 653–672, 2022.
Abstract | Links | BibTeX | Tags: Machine learning potential, Review
@article{Fedik2022,
title = {Extending machine learning beyond interatomic potentials for predicting molecular properties},
author = {Nikita Fedik and Roman Zubatyuk and Maksim Kulichenko and Nicholas Lubbers and Justin S. Smith and Benjamin Nebgen and Richard Messerly and Ying Wai Li and Alexander I. Boldyrev and Kipton Barros and Olexandr Isayev and Sergei Tretiak},
doi = {10.1038/s41570-022-00416-3},
year = {2022},
date = {2022-10-14},
urldate = {2022-10-14},
journal = {Nat Rev Chem},
volume = {6},
number = {9},
pages = {653--672},
publisher = {Springer Science and Business Media LLC},
abstract = {Machine learning (ML) is becoming a method of choice for modelling complex chemical processes and materials. ML provides a surrogate model trained on a reference dataset that can be used to establish a relationship between a molecular structure and its chemical properties. This Review highlights developments in the use of ML to evaluate chemical properties such as partial atomic charges, dipole moments, spin and electron densities, and chemical bonding, as well as to obtain a reduced quantum-mechanical description. We overview several modern neural network architectures, their predictive capabilities, generality and transferability, and illustrate their applicability to various chemical properties. We emphasize that learned molecular representations resemble quantum-mechanical analogues, demonstrating the ability of the models to capture the underlying physics. We also discuss how ML models can describe non-local quantum effects. Finally, we conclude by compiling a list of available ML toolboxes, summarizing the unresolved challenges and presenting an outlook for future development. The observed trends demonstrate that this field is evolving towards physics-based models augmented by ML, which is accompanied by the development of new methods and the rapid growth of user-friendly ML frameworks for chemistry.},
keywords = {Machine learning potential, Review},
pubstate = {published},
tppubtype = {article}
}
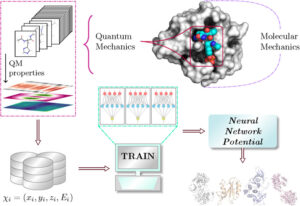
Gokcan, Hatice; Isayev, Olexandr
Learning molecular potentials with neural networks Journal Article
In: WIREs Comput Mol Sci, vol. 12, no. 2, pp. e1564, 2022.
Abstract | Links | BibTeX | Tags: ANI, Machine learning potential, Review
@article{Gokcan2021,
title = {Learning molecular potentials with neural networks},
author = {Hatice Gokcan and Olexandr Isayev},
doi = {10.1002/wcms.1564},
year = {2022},
date = {2022-07-14},
journal = {WIREs Comput Mol Sci},
volume = {12},
number = {2},
pages = {e1564},
publisher = {Wiley},
abstract = {\<jats:title\>Abstract\</jats:title\>\<jats:p\>The potential energy of molecular species and their conformers can be computed with a wide range of computational chemistry methods, from molecular mechanics to ab initio quantum chemistry. However, the proper choice of the computational approach based on computational cost and reliability of calculated energies is a dilemma, especially for large molecules. This dilemma is proved to be even more problematic for studies that require hundreds and thousands of calculations, such as drug discovery. On the other hand, driven by their pattern recognition capabilities, neural networks started to gain popularity in the computational chemistry community. During the last decade, many neural network potentials have been developed to predict a variety of chemical information of different systems. Neural network potentials are proved to predict chemical properties with accuracy comparable to quantum mechanical approaches but with the cost approaching molecular mechanics calculations. As a result, the development of more reliable, transferable, and extensible neural network potentials became an attractive field of study for researchers. In this review, we outlined an overview of the status of current neural network potentials and strategies to improve their accuracy. We provide recent examples of studies that prove the applicability of these potentials. We also discuss the capabilities and shortcomings of the current models and the challenges and future aspects of their development and applications. It is expected that this review would provide guidance for the development of neural network potentials and the exploitation of their applicability.\</jats:p\>\<jats:p\>This article is categorized under:\<jats:list list-type="simple"\>\<jats:list-item\>\<jats:p\>Data Science \> Artificial Intelligence/Machine Learning\</jats:p\>\</jats:list-item\>\<jats:list-item\>\<jats:p\>Molecular and Statistical Mechanics \> Molecular Interactions\</jats:p\>\</jats:list-item\>\<jats:list-item\>\<jats:p\>Software \> Molecular Modeling\</jats:p\>\</jats:list-item\>\</jats:list\>\</jats:p\>},
keywords = {ANI, Machine learning potential, Review},
pubstate = {published},
tppubtype = {article}
}
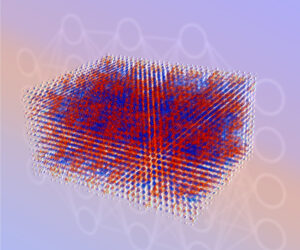
Kulik, H J; Hammerschmidt, T; Schmidt, J; Botti, S; Marques, M A L; Boley, M; Scheffler, M; Todorović, M; Rinke, P; Oses, C; Smolyanyuk, A; Curtarolo, S; Tkatchenko, A; Bartók, A P; Manzhos, S; Ihara, M; Carrington, T; Behler, J; Isayev, O; Veit, M; Grisafi, A; Nigam, J; Ceriotti, M; Schütt, K T; Westermayr, J; Gastegger, M; Maurer, R J; Kalita, B; Burke, K; Nagai, R; Akashi, R; Sugino, O; Hermann, J; Noé, F; Pilati, S; Draxl, C; Kuban, M; Rigamonti, S; Scheidgen, M; Esters, M; Hicks, D; Toher, C; Balachandran, P V; Tamblyn, I; Whitelam, S; Bellinger, C; Ghiringhelli, L M
Roadmap on Machine learning in electronic structure Journal Article
In: Electron. Struct., vol. 4, no. 2, pp. 023004, 2022.
Abstract | Links | BibTeX | Tags: Machine learning potential, Materials informatics, Review
@article{Kulik2022,
title = {Roadmap on Machine learning in electronic structure},
author = {H J Kulik and T Hammerschmidt and J Schmidt and S Botti and M A L Marques and M Boley and M Scheffler and M Todorovi\'{c} and P Rinke and C Oses and A Smolyanyuk and S Curtarolo and A Tkatchenko and A P Bart\'{o}k and S Manzhos and M Ihara and T Carrington and J Behler and O Isayev and M Veit and A Grisafi and J Nigam and M Ceriotti and K T Sch\"{u}tt and J Westermayr and M Gastegger and R J Maurer and B Kalita and K Burke and R Nagai and R Akashi and O Sugino and J Hermann and F No\'{e} and S Pilati and C Draxl and M Kuban and S Rigamonti and M Scheidgen and M Esters and D Hicks and C Toher and P V Balachandran and I Tamblyn and S Whitelam and C Bellinger and L M Ghiringhelli},
doi = {10.1088/2516-1075/ac572f},
year = {2022},
date = {2022-06-01},
urldate = {2022-06-01},
journal = {Electron. Struct.},
volume = {4},
number = {2},
pages = {023004},
publisher = {IOP Publishing},
abstract = {\<jats:title\>Abstract\</jats:title\>\<jats:p\>In recent years, we have been witnessing a paradigm shift in computational materials science. In fact, traditional methods, mostly developed in the second half of the XXth century, are being complemented, extended, and sometimes even completely replaced by faster, simpler, and often more accurate approaches. The new approaches, that we collectively label by machine learning, have their origins in the fields of informatics and artificial intelligence, but are making rapid inroads in all other branches of science. With this in mind, this Roadmap article, consisting of multiple contributions from experts across the field, discusses the use of machine learning in materials science, and share perspectives on current and future challenges in problems as diverse as the prediction of materials properties, the construction of force-fields, the development of exchange correlation functionals for density-functional theory, the solution of the many-body problem, and more. In spite of the already numerous and exciting success stories, we are just at the beginning of a long path that will reshape materials science for the many challenges of the XXIth century.\</jats:p\>},
keywords = {Machine learning potential, Materials informatics, Review},
pubstate = {published},
tppubtype = {article}
}
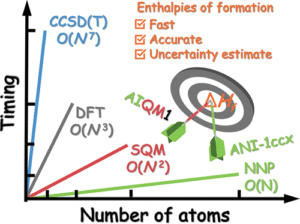
Zheng, Peikun; Yang, Wudi; Wu, Wei; Isayev, Olexandr; Dral, Pavlo O.
Toward Chemical Accuracy in Predicting Enthalpies of Formation with General-Purpose Data-Driven Methods Journal Article
In: J. Phys. Chem. Lett., vol. 13, no. 15, pp. 3479–3491, 2022.
Abstract | Links | BibTeX | Tags: ANI, Machine learning potential
@article{Zheng2022,
title = {Toward Chemical Accuracy in Predicting Enthalpies of Formation with General-Purpose Data-Driven Methods},
author = {Peikun Zheng and Wudi Yang and Wei Wu and Olexandr Isayev and Pavlo O. Dral},
doi = {10.1021/acs.jpclett.2c00734},
year = {2022},
date = {2022-04-21},
urldate = {2022-04-21},
journal = {J. Phys. Chem. Lett.},
volume = {13},
number = {15},
pages = {3479--3491},
publisher = {American Chemical Society (ACS)},
abstract = {Enthalpies of formation and reaction are important thermodynamic properties that have a crucial impact on the outcome of chemical transformations. Here we implement the calculation of enthalpies of formation with a general-purpose ANI-1ccx neural network atomistic potential. We demonstrate on a wide range of benchmark sets that both ANI-1ccx and our other general-purpose data-driven method AIQM1 approach the coveted chemical accuracy of 1 kcal/mol with the speed of semiempirical quantum mechanical methods (AIQM1) or faster (ANI-1ccx). It is remarkably achieved without specifically training the machine learning parts of ANI-1ccx or AIQM1 on formation enthalpies. Importantly, we show that these data-driven methods provide statistical means for uncertainty quantification of their predictions, which we use to detect and eliminate outliers and revise reference experimental data. Uncertainty quantification may also help in the systematic improvement of such data-driven methods.},
keywords = {ANI, Machine learning potential},
pubstate = {published},
tppubtype = {article}
}
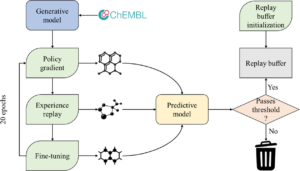
Korshunova, Maria; Huang, Niles; Capuzzi, Stephen; Radchenko, Dmytro S.; Savych, Olena; Moroz, Yuriy S.; Wells, Carrow I.; Willson, Timothy M.; Tropsha, Alexander; Isayev, Olexandr
Generative and reinforcement learning approaches for the automated de novo design of bioactive compounds Journal Article
In: Commun Chem, vol. 5, no. 1, pp. 129 , 2022.
Abstract | Links | BibTeX | Tags: Drug Discovery, Generative AI, RL
@article{Korshunova2022,
title = {Generative and reinforcement learning approaches for the automated de novo design of bioactive compounds},
author = {Maria Korshunova and Niles Huang and Stephen Capuzzi and Dmytro S. Radchenko and Olena Savych and Yuriy S. Moroz and Carrow I. Wells and Timothy M. Willson and Alexander Tropsha and Olexandr Isayev},
doi = {10.1038/s42004-022-00733-0},
year = {2022},
date = {2022-03-31},
urldate = {2022-03-31},
journal = {Commun Chem},
volume = {5},
number = {1},
pages = {129 },
publisher = {Springer Science and Business Media LLC},
abstract = {\<jats:title\>Abstract\</jats:title\>\<jats:p\>Deep generative neural networks have been used increasingly in computational chemistry for \<jats:italic\>de novo\</jats:italic\> design of molecules with desired properties. Many deep learning approaches employ reinforcement learning for optimizing the target properties of the generated molecules. However, the success of this approach is often hampered by the problem of sparse rewards as the majority of the generated molecules are expectedly predicted as inactives. We propose several technical innovations to address this problem and improve the balance between exploration and exploitation modes in reinforcement learning. In a proof-of-concept study, we demonstrate the application of the deep generative recurrent neural network architecture enhanced by several proposed technical tricks to design inhibitors of the epidermal growth factor (EGFR) and further experimentally validate their potency. The proposed technical solutions are expected to substantially improve the success rate of finding novel bioactive compounds for specific biological targets using generative and reinforcement learning approaches.\</jats:p\>},
keywords = {Drug Discovery, Generative AI, RL},
pubstate = {published},
tppubtype = {article}
}
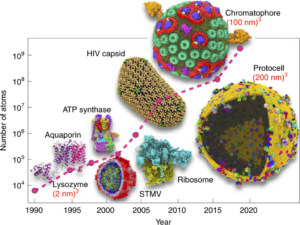
Pandey, Mohit; Fernandez, Michael; Gentile, Francesco; Isayev, Olexandr; Tropsha, Alexander; Stern, Abraham C.; Cherkasov, Artem
The transformational role of GPU computing and deep learning in drug discovery Journal Article
In: Nat Mach Intell, vol. 4, no. 3, pp. 211–221, 2022.
Abstract | Links | BibTeX | Tags: Drug Discovery, Review
@article{Pandey2022,
title = {The transformational role of GPU computing and deep learning in drug discovery},
author = {Mohit Pandey and Michael Fernandez and Francesco Gentile and Olexandr Isayev and Alexander Tropsha and Abraham C. Stern and Artem Cherkasov},
doi = {10.1038/s42256-022-00463-x},
year = {2022},
date = {2022-03-04},
urldate = {2022-03-04},
journal = {Nat Mach Intell},
volume = {4},
number = {3},
pages = {211--221},
publisher = {Springer Science and Business Media LLC},
abstract = {Deep learning has disrupted nearly every field of research, including those of direct importance to drug discovery, such as medicinal chemistry and pharmacology. This revolution has largely been attributed to the unprecedented advances in highly parallelizable graphics processing units (GPUs) and the development of GPU-enabled algorithms. In this Review, we present a comprehensive overview of historical trends and recent advances in GPU algorithms and discuss their immediate impact on the discovery of new drugs and drug targets. We also cover the state-of-the-art of deep learning architectures that have found practical applications in both early drug discovery and consequent hit-to-lead optimization stages, including the acceleration of molecular docking, the evaluation of off-target effects and the prediction of pharmacological properties. We conclude by discussing the impacts of GPU acceleration and deep learning models on the global democratization of the field of drug discovery that may lead to efficient exploration of the ever-expanding chemical universe to accelerate the discovery of novel medicines.},
keywords = {Drug Discovery, Review},
pubstate = {published},
tppubtype = {article}
}
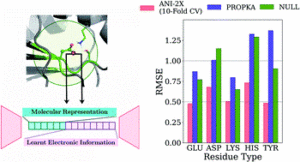
Gokcan, Hatice; Isayev, Olexandr
Prediction of protein pKawith representation learning Journal Article
In: Chem. Sci., vol. 13, no. 8, pp. 2462–2474, 2022.
Abstract | Links | BibTeX | Tags: ANI, Drug Discovery
@article{Gokcan2022,
title = {Prediction of protein p\textit{K}_{a}with representation learning},
author = {Hatice Gokcan and Olexandr Isayev},
doi = {10.1039/d1sc05610g},
year = {2022},
date = {2022-02-23},
urldate = {2022-02-23},
journal = {Chem. Sci.},
volume = {13},
number = {8},
pages = {2462--2474},
publisher = {Royal Society of Chemistry (RSC)},
abstract = {\<jats:p\>We developed new empirical ML model for protein p\<jats:italic\>K\</jats:italic\>\<jats:sub\>a\</jats:sub\>prediction with MAEs below 0.5 for all amino acid types.\</jats:p\>},
keywords = {ANI, Drug Discovery},
pubstate = {published},
tppubtype = {article}
}
2021
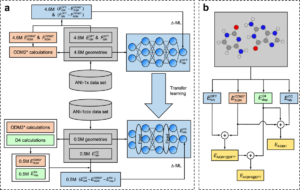
Zheng, Peikun; Zubatyuk, Roman; Wu, Wei; Isayev, Olexandr; Dral, Pavlo O.
Artificial intelligence-enhanced quantum chemical method with broad applicability Journal Article
In: Nat Commun, vol. 12, pp. 7022 , 2021, ISSN: 2041-1723.
Abstract | Links | BibTeX | Tags: ANI, Machine learning potential
@article{Zheng2021,
title = {Artificial intelligence-enhanced quantum chemical method with broad applicability},
author = {Peikun Zheng and Roman Zubatyuk and Wei Wu and Olexandr Isayev and Pavlo O. Dral},
doi = {10.1038/s41467-021-27340-2},
issn = {2041-1723},
year = {2021},
date = {2021-12-15},
urldate = {2021-12-15},
journal = {Nat Commun},
volume = {12},
pages = {7022 },
publisher = {Springer Science and Business Media LLC},
abstract = {High-level quantum mechanical (QM) calculations are indispensable for accurate explanation of natural phenomena on the atomistic level. Their staggering computational cost, however, poses great limitations, which luckily can be lifted to a great extent by exploiting advances in artificial intelligence (AI). Here we introduce the general-purpose, highly transferable artificial intelligence\textendashquantum mechanical method 1 (AIQM1). It approaches the accuracy of the gold-standard coupled cluster QM method with high computational speed of the approximate low-level semiempirical QM methods for the neutral, closed-shell species in the ground state. AIQM1 can provide accurate ground-state energies for diverse organic compounds as well as geometries for even challenging systems such as large conjugated compounds (fullerene C\<jats:sub\>60\</jats:sub\>) close to experiment. This opens an opportunity to investigate chemical compounds with previously unattainable speed and accuracy as we demonstrate by determining geometries of polyyne molecules\textemdashthe task difficult for both experiment and theory. Noteworthy, our method’s accuracy is also good for ions and excited-state properties, although the neural network part of AIQM1 was never fitted to these properties.},
keywords = {ANI, Machine learning potential},
pubstate = {published},
tppubtype = {article}
}
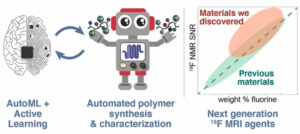
Reis, Marcus; Gusev, Filipp; Taylor, Nicholas G.; Chung, Sang Hun; Verber, Matthew D.; Lee, Yueh Z.; Isayev, Olexandr; Leibfarth, Frank A.
Machine-Learning-Guided Discovery of 19F MRI Agents Enabled by Automated Copolymer Synthesis Journal Article
In: J. Am. Chem. Soc., vol. 143, no. 42, pp. 17677–17689, 2021, ISSN: 1520-5126.
Abstract | Links | BibTeX | Tags: Materials informatics, Science automation
@article{Reis2021,
title = {Machine-Learning-Guided Discovery of ^{19}F MRI Agents Enabled by Automated Copolymer Synthesis},
author = {Marcus Reis and Filipp Gusev and Nicholas G. Taylor and Sang Hun Chung and Matthew D. Verber and Yueh Z. Lee and Olexandr Isayev and Frank A. Leibfarth},
doi = {10.1021/jacs.1c08181},
issn = {1520-5126},
year = {2021},
date = {2021-10-27},
urldate = {2021-10-27},
journal = {J. Am. Chem. Soc.},
volume = {143},
number = {42},
pages = {17677--17689},
publisher = {American Chemical Society (ACS)},
abstract = {Modern polymer science suffers from the curse of multidimensionality. The large chemical space imposed by including combinations of monomers into a statistical copolymer overwhelms polymer synthesis and characterization technology and limits the ability to systematically study structure\textendashproperty relationships. To tackle this challenge in the context of 19F magnetic resonance imaging (MRI) agents, we pursued a computer-guided materials discovery approach that combines synergistic innovations in automated flow synthesis and machine learning (ML) method development. A software-controlled, continuous polymer synthesis platform was developed to enable iterative experimental\textendashcomputational cycles that resulted in the synthesis of 397 unique copolymer compositions within a six-variable compositional space. The nonintuitive design criteria identified by ML, which were accomplished by exploring \<0.9% of the overall compositional space, lead to the identification of \>10 copolymer compositions that outperformed state-of-the-art materials.},
keywords = {Materials informatics, Science automation},
pubstate = {published},
tppubtype = {article}
}
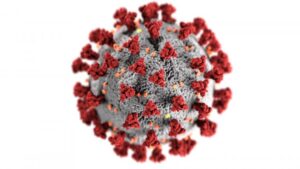
Muratov, Eugene N.; Amaro, Rommie; Andrade, Carolina H.; Brown, Nathan; Ekins, Sean; Fourches, Denis; Isayev, Olexandr; Kozakov, Dima; Medina-Franco, José L.; Merz, Kenneth M.; Oprea, Tudor I.; Poroikov, Vladimir; Schneider, Gisbert; Todd, Matthew H.; Varnek, Alexandre; Winkler, David A.; Zakharov, Alexey V.; Cherkasov, Artem; Tropsha, Alexander
A critical overview of computational approaches employed for COVID-19 drug discovery Journal Article
In: Chem. Soc. Rev., vol. 50, no. 16, pp. 9121–9151, 2021, ISSN: 1460-4744.
Abstract | Links | BibTeX | Tags: COVID19
@article{Muratov2021,
title = {A critical overview of computational approaches employed for COVID-19 drug discovery},
author = {Eugene N. Muratov and Rommie Amaro and Carolina H. Andrade and Nathan Brown and Sean Ekins and Denis Fourches and Olexandr Isayev and Dima Kozakov and Jos\'{e} L. Medina-Franco and Kenneth M. Merz and Tudor I. Oprea and Vladimir Poroikov and Gisbert Schneider and Matthew H. Todd and Alexandre Varnek and David A. Winkler and Alexey V. Zakharov and Artem Cherkasov and Alexander Tropsha},
doi = {10.1039/d0cs01065k},
issn = {1460-4744},
year = {2021},
date = {2021-08-16},
urldate = {2021-08-16},
journal = {Chem. Soc. Rev.},
volume = {50},
number = {16},
pages = {9121--9151},
publisher = {Royal Society of Chemistry (RSC)},
abstract = {\<jats:p\>We cover diverse methodologies, computational approaches, and case studies illustrating the ongoing efforts to develop viable drug candidates for treatment of COVID-19.\</jats:p\>},
keywords = {COVID19},
pubstate = {published},
tppubtype = {article}
}
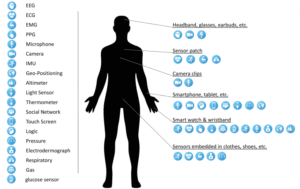
Firouzi, Farshad; Farahani, Bahar; Daneshmand, Mahmoud; Grise, Kathy; Song, Jaeseung; Saracco, Roberto; Wang, Lucy Lu; Lo, Kyle; Angelov, Plamen; Soares, Eduardo; Loh, Po-Shen; Talebpour, Zeynab; Moradi, Reza; Goodarzi, Mohsen; Ashraf, Haleh; Talebpour, Mohammad; Talebpour, Alireza; Romeo, Luca; Das, Rupam; Heidari, Hadi; Pasquale, Dana; Moody, James; Woods, Chris; Huang, Erich S.; Barnaghi, Payam; Sarrafzadeh, Majid; Li, Ron; Beck, Kristen L.; Isayev, Olexandr; Sung, Nakmyoung; Luo, Alan
Harnessing the Power of Smart and Connected Health to Tackle COVID-19: IoT, AI, Robotics, and Blockchain for a Better World Journal Article
In: IEEE Internet Things J., vol. 8, no. 16, pp. 12826–12846, 2021, ISSN: 2327-4662.
Abstract | Links | BibTeX | Tags: COVID19
@article{Firouzi2021,
title = {Harnessing the Power of Smart and Connected Health to Tackle COVID-19: IoT, AI, Robotics, and Blockchain for a Better World},
author = {Farshad Firouzi and Bahar Farahani and Mahmoud Daneshmand and Kathy Grise and Jaeseung Song and Roberto Saracco and Lucy Lu Wang and Kyle Lo and Plamen Angelov and Eduardo Soares and Po-Shen Loh and Zeynab Talebpour and Reza Moradi and Mohsen Goodarzi and Haleh Ashraf and Mohammad Talebpour and Alireza Talebpour and Luca Romeo and Rupam Das and Hadi Heidari and Dana Pasquale and James Moody and Chris Woods and Erich S. Huang and Payam Barnaghi and Majid Sarrafzadeh and Ron Li and Kristen L. Beck and Olexandr Isayev and Nakmyoung Sung and Alan Luo},
doi = {10.1109/jiot.2021.3073904},
issn = {2327-4662},
year = {2021},
date = {2021-08-15},
urldate = {2021-08-15},
journal = {IEEE Internet Things J.},
volume = {8},
number = {16},
pages = {12826--12846},
publisher = {Institute of Electrical and Electronics Engineers (IEEE)},
abstract = {As COVID-19 hounds the world, the common cause of finding a swift solution to manage the pandemic has brought together researchers, institutions, governments, and society at large. The Internet of Things (IoT), artificial intelligence (AI)\textemdashincluding machine learning (ML) and Big Data analytics\textemdashas well as Robotics and Blockchain, are the four decisive areas of technological innovation that have been ingenuity harnessed to fight this pandemic and future ones. While these highly interrelated smart and connected health technologies cannot resolve the pandemic overnight and may not be the only answer to the crisis, they can provide greater insight into the disease and support frontline efforts to prevent and control the pandemic. This article provides a blend of discussions on the contribution of these digital technologies, propose several complementary and multidisciplinary techniques to combat COVID-19, offer opportunities for more holistic studies, and accelerate knowledge acquisition and scientific discoveries in pandemic research. First, four areas, where IoT can contribute are discussed, namely: 1) tracking and tracing; 2) remote patient monitoring (RPM) by wearable IoT (WIoT); 3) personal digital twins (PDTs); and 4) real-life use case: ICT/IoT solution in South Korea. Second, the role and novel applications of AI are explained, namely: 1) diagnosis and prognosis; 2) risk prediction; 3) vaccine and drug development; 4) research data set; 5) early warnings and alerts; 6) social control and fake news detection; and 7) communication and chatbot. Third, the main uses of robotics and drone technology are analyzed, including: 1) crowd surveillance; 2) public announcements; 3) screening and diagnosis; and 4) essential supply delivery. Finally, we discuss how distributed ledger technologies (DLTs), of which blockchain is a common example, can be combined with other technologies for tackling COVID-19.},
keywords = {COVID19},
pubstate = {published},
tppubtype = {article}
}
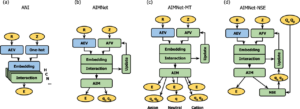
Zubatyuk, Roman; Smith, Justin S.; Nebgen, Benjamin T.; Tretiak, Sergei; Isayev, Olexandr
Teaching a neural network to attach and detach electrons from molecules Journal Article
In: Nat Commun, vol. 12, no. 1, 2021, ISSN: 2041-1723.
Abstract | Links | BibTeX | Tags: AIMNet, Machine learning potential
@article{Zubatyuk2021,
title = {Teaching a neural network to attach and detach electrons from molecules},
author = {Roman Zubatyuk and Justin S. Smith and Benjamin T. Nebgen and Sergei Tretiak and Olexandr Isayev},
doi = {10.1038/s41467-021-24904-0},
issn = {2041-1723},
year = {2021},
date = {2021-08-11},
journal = {Nat Commun},
volume = {12},
number = {1},
publisher = {Springer Science and Business Media LLC},
abstract = {Interatomic potentials derived with Machine Learning algorithms such as Deep-Neural Networks (DNNs), achieve the accuracy of high-fidelity quantum mechanical (QM) methods in areas traditionally dominated by empirical force fields and allow performing massive simulations. Most DNN potentials were parametrized for neutral molecules or closed-shell ions due to architectural limitations. In this work, we propose an improved machine learning framework for simulating open-shell anions and cations. We introduce the AIMNet-NSE (Neural Spin Equilibration) architecture, which can predict molecular energies for an arbitrary combination of molecular charge and spin multiplicity with errors of about 2\textendash3 kcal/mol and spin-charges with error errors ~0.01e for small and medium-sized organic molecules, compared to the reference QM simulations. The AIMNet-NSE model allows to fully bypass QM calculations and derive the ionization potential, electron affinity, and conceptual Density Functional Theory quantities like electronegativity, hardness, and condensed Fukui functions. We show that these descriptors, along with learned atomic representations, could be used to model chemical reactivity through an example of regioselectivity in electrophilic aromatic substitution reactions.},
keywords = {AIMNet, Machine learning potential},
pubstate = {published},
tppubtype = {article}
}
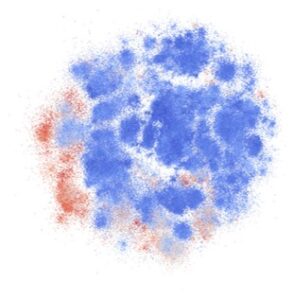
Fronzi, Marco; Isayev, Olexandr; Winkler, David A.; Shapter, Joseph G.; Ellis, Amanda V.; Sherrell, Peter C.; Shepelin, Nick A.; Corletto, Alexander; Ford, Michael J.
Active Learning in Bayesian Neural Networks for Bandgap Predictions of Novel Van der Waals Heterostructures Journal Article
In: Advanced Intelligent Systems, vol. 3, no. 11, 2021, ISSN: 2640-4567.
Abstract | Links | BibTeX | Tags: Materials informatics
@article{Fronzi2021,
title = {Active Learning in Bayesian Neural Networks for Bandgap Predictions of Novel Van der Waals Heterostructures},
author = {Marco Fronzi and Olexandr Isayev and David A. Winkler and Joseph G. Shapter and Amanda V. Ellis and Peter C. Sherrell and Nick A. Shepelin and Alexander Corletto and Michael J. Ford},
doi = {10.1002/aisy.202100080},
issn = {2640-4567},
year = {2021},
date = {2021-08-02},
journal = {Advanced Intelligent Systems},
volume = {3},
number = {11},
publisher = {Wiley},
abstract = {\<jats:sec\>\<jats:label /\>\<jats:p\>The bandgap is one of the most fundamental properties of condensed matter. However, an accurate calculation of its value, which could potentially allow experimentalists to identify materials suitable for device applications, is very computationally expensive. Here, active machine learning algorithms are used to leverage a limited number of accurate density functional theory calculations to robustly predict the bandgap of a very large number of novel 2D heterostructures. Using this approach, a database of ≈2.2 million bandgap values for various novel 2D van der Waals heterostructures is produced.\</jats:p\>\</jats:sec\>},
keywords = {Materials informatics},
pubstate = {published},
tppubtype = {article}
}
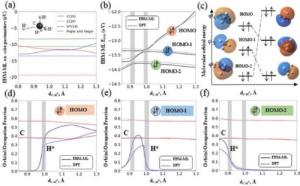
Zubatiuk, Tetiana; Nebgen, Benjamin; Lubbers, Nicholas; Smith, Justin S.; Zubatyuk, Roman; Zhou, Guoqing; Koh, Christopher; Barros, Kipton; Isayev, Olexandr; Tretiak, Sergei
Machine learned Hückel theory: Interfacing physics and deep neural networks Journal Article
In: vol. 154, no. 24, 2021, ISSN: 1089-7690.
Abstract | Links | BibTeX | Tags: Machine learning potential
@article{Zubatiuk2021b,
title = {Machine learned H\"{u}ckel theory: Interfacing physics and deep neural networks},
author = {Tetiana Zubatiuk and Benjamin Nebgen and Nicholas Lubbers and Justin S. Smith and Roman Zubatyuk and Guoqing Zhou and Christopher Koh and Kipton Barros and Olexandr Isayev and Sergei Tretiak},
doi = {10.1063/5.0052857},
issn = {1089-7690},
year = {2021},
date = {2021-06-28},
urldate = {2021-06-28},
volume = {154},
number = {24},
publisher = {AIP Publishing},
abstract = {\<jats:p\>The H\"{u}ckel Hamiltonian is an incredibly simple tight-binding model known for its ability to capture qualitative physics phenomena arising from electron interactions in molecules and materials. Part of its simplicity arises from using only two types of empirically fit physics-motivated parameters: the first describes the orbital energies on each atom and the second describes electronic interactions and bonding between atoms. By replacing these empirical parameters with machine-learned dynamic values, we vastly increase the accuracy of the extended H\"{u}ckel model. The dynamic values are generated with a deep neural network, which is trained to reproduce orbital energies and densities derived from density functional theory. The resulting model retains interpretability, while the deep neural network parameterization is smooth and accurate and reproduces insightful features of the original empirical parameterization. Overall, this work shows the promise of utilizing machine learning to formulate simple, accurate, and dynamically parameterized physics models.\</jats:p\>},
keywords = {Machine learning potential},
pubstate = {published},
tppubtype = {article}
}
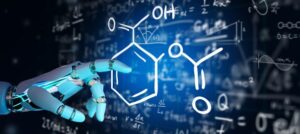
Artrith, Nongnuch; Butler, Keith T.; Coudert, François-Xavier; Han, Seungwu; Isayev, Olexandr; Jain, Anubhav; Walsh, Aron
Best practices in machine learning for chemistry Journal Article
In: Nat. Chem., vol. 13, no. 6, pp. 505–508, 2021, ISSN: 1755-4349.
Abstract | Links | BibTeX | Tags: Machine learning potential, Review
@article{Artrith2021,
title = {Best practices in machine learning for chemistry},
author = {Nongnuch Artrith and Keith T. Butler and Fran\c{c}ois-Xavier Coudert and Seungwu Han and Olexandr Isayev and Anubhav Jain and Aron Walsh},
doi = {10.1038/s41557-021-00716-z},
issn = {1755-4349},
year = {2021},
date = {2021-06-15},
urldate = {2021-06-15},
journal = {Nat. Chem.},
volume = {13},
number = {6},
pages = {505--508},
publisher = {Springer Science and Business Media LLC},
abstract = {Statistical tools based on machine learning are becoming integrated into chemistry research workflows. We discuss the elements necessary to train reliable, repeatable and reproducible models, and recommend a set of guidelines for machine learning reports.},
keywords = {Machine learning potential, Review},
pubstate = {published},
tppubtype = {article}
}
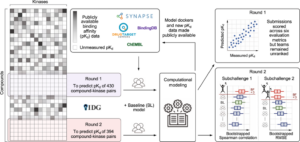
Cichońska, Anna; Ravikumar, Balaguru; Allaway, Robert J.; Wan, Fangping; Park, Sungjoon; Isayev, Olexandr; Li, Shuya; Mason, Michael; Lamb, Andrew; Tanoli, Ziaurrehman; Jeon, Minji; Kim, Sunkyu; Popova, Mariya; Capuzzi, Stephen; Zeng, Jianyang; Dang, Kristen; Koytiger, Gregory; Kang, Jaewoo; Wells, Carrow I.; Willson, Timothy M.; Tan, Mehmet; Huang, Chih-Han; Shih, Edward S. C.; Chen, Tsai-Min; Wu, Chih-Hsun; Fang, Wei-Quan; Chen, Jhih-Yu; Hwang, Ming-Jing; Wang, Xiaokang; Guebila, Marouen Ben; Shamsaei, Behrouz; Singh, Sourav; Nguyen, Thin; Karimi, Mostafa; Wu, Di; Wang, Zhangyang; Shen, Yang; Öztürk, Hakime; Ozkirimli, Elif; Özgür, Arzucan; Lim, Hansaim; Xie, Lei; Kanev, Georgi K.; Kooistra, Albert J.; Westerman, Bart A.; Terzopoulos, Panagiotis; Ntagiantas, Konstantinos; Fotis, Christos; Alexopoulos, Leonidas; Boeckaerts, Dimitri; Stock, Michiel; Baets, Bernard De; Briers, Yves; Luo, Yunan; Hu, Hailin; Peng, Jian; Dogan, Tunca; Rifaioglu, Ahmet S.; Atas, Heval; Atalay, Rengul Cetin; Atalay, Volkan; Martin, Maria J.; Jeon, Minji; Lee, Junhyun; Yun, Seongjun; Kim, Bumsoo; Chang, Buru; Turu, Gábor; Misák, Ádám; Szalai, Bence; Hunyady, László; Lienhard, Matthias; Prasse, Paul; Bachmann, Ivo; Ganzlin, Julia; Barel, Gal; Herwig, Ralf; Oršolić, Davor; Lučić, Bono; Stepanić, Višnja; Šmuc, Tomislav; Oprea, Tudor I.; Schlessinger, Avner; Drewry, David H.; Stolovitzky, Gustavo; Wennerberg, Krister; Guinney, Justin; Aittokallio, Tero
Crowdsourced mapping of unexplored target space of kinase inhibitors Journal Article
In: Nat Commun, vol. 12, pp. 3307 , 2021.
Abstract | Links | BibTeX | Tags: Drug Discovery
@article{Cicho\'{n}ska2021,
title = {Crowdsourced mapping of unexplored target space of kinase inhibitors},
author = {Anna Cicho\'{n}ska and Balaguru Ravikumar and Robert J. Allaway and Fangping Wan and Sungjoon Park and Olexandr Isayev and Shuya Li and Michael Mason and Andrew Lamb and Ziaurrehman Tanoli and Minji Jeon and Sunkyu Kim and Mariya Popova and Stephen Capuzzi and Jianyang Zeng and Kristen Dang and Gregory Koytiger and Jaewoo Kang and Carrow I. Wells and Timothy M. Willson and Mehmet Tan and Chih-Han Huang and Edward S. C. Shih and Tsai-Min Chen and Chih-Hsun Wu and Wei-Quan Fang and Jhih-Yu Chen and Ming-Jing Hwang and Xiaokang Wang and Marouen Ben Guebila and Behrouz Shamsaei and Sourav Singh and Thin Nguyen and Mostafa Karimi and Di Wu and Zhangyang Wang and Yang Shen and Hakime \"{O}zt\"{u}rk and Elif Ozkirimli and Arzucan \"{O}zg\"{u}r and Hansaim Lim and Lei Xie and Georgi K. Kanev and Albert J. Kooistra and Bart A. Westerman and Panagiotis Terzopoulos and Konstantinos Ntagiantas and Christos Fotis and Leonidas Alexopoulos and Dimitri Boeckaerts and Michiel Stock and Bernard De Baets and Yves Briers and Yunan Luo and Hailin Hu and Jian Peng and Tunca Dogan and Ahmet S. Rifaioglu and Heval Atas and Rengul Cetin Atalay and Volkan Atalay and Maria J. Martin and Minji Jeon and Junhyun Lee and Seongjun Yun and Bumsoo Kim and Buru Chang and G\'{a}bor Turu and \'{A}d\'{a}m Mis\'{a}k and Bence Szalai and L\'{a}szl\'{o} Hunyady and Matthias Lienhard and Paul Prasse and Ivo Bachmann and Julia Ganzlin and Gal Barel and Ralf Herwig and Davor Or\v{s}oli\'{c} and Bono Lu\v{c}i\'{c} and Vi\v{s}nja Stepani\'{c} and Tomislav \v{S}muc and Tudor I. Oprea and Avner Schlessinger and David H. Drewry and Gustavo Stolovitzky and Krister Wennerberg and Justin Guinney and Tero Aittokallio},
doi = {10.1038/s41467-021-23165-1},
year = {2021},
date = {2021-06-04},
urldate = {2021-06-04},
journal = {Nat Commun},
volume = {12},
pages = {3307 },
publisher = {Springer Science and Business Media LLC},
abstract = {Despite decades of intensive search for compounds that modulate the activity of particular protein targets, a large proportion of the human kinome remains as yet undrugged. Effective approaches are therefore required to map the massive space of unexplored compound\textendashkinase interactions for novel and potent activities. Here, we carry out a crowdsourced benchmarking of predictive algorithms for kinase inhibitor potencies across multiple kinase families tested on unpublished bioactivity data. We find the top-performing predictions are based on various models, including kernel learning, gradient boosting and deep learning, and their ensemble leads to a predictive accuracy exceeding that of single-dose kinase activity assays. We design experiments based on the model predictions and identify unexpected activities even for under-studied kinases, thereby accelerating experimental mapping efforts. The open-source prediction algorithms together with the bioactivities between 95 compounds and 295 kinases provide a resource for benchmarking prediction algorithms and for extending the druggable kinome.},
keywords = {Drug Discovery},
pubstate = {published},
tppubtype = {article}
}
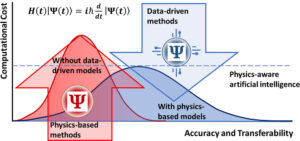
Zubatiuk, Tetiana; Isayev, Olexandr
Development of Multimodal Machine Learning Potentials: Toward a Physics-Aware Artificial Intelligence Journal Article
In: Acc. Chem. Res., vol. 54, no. 7, pp. 1575–1585, 2021, ISSN: 1520-4898.
Links | BibTeX | Tags: AIMNet, Machine learning potential
@article{Zubatiuk2021,
title = {Development of Multimodal Machine Learning Potentials: Toward a Physics-Aware Artificial Intelligence},
author = {Tetiana Zubatiuk and Olexandr Isayev},
doi = {10.1021/acs.accounts.0c00868},
issn = {1520-4898},
year = {2021},
date = {2021-04-06},
urldate = {2021-04-06},
journal = {Acc. Chem. Res.},
volume = {54},
number = {7},
pages = {1575--1585},
publisher = {American Chemical Society (ACS)},
keywords = {AIMNet, Machine learning potential},
pubstate = {published},
tppubtype = {article}
}
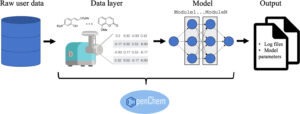
Korshunova, Maria; Ginsburg, Boris; Tropsha, Alexander; Isayev, Olexandr
OpenChem: A Deep Learning Toolkit for Computational Chemistry and Drug Design Journal Article
In: J. Chem. Inf. Model., vol. 61, no. 1, pp. 7–13, 2021, ISSN: 1549-960X.
Abstract | Links | BibTeX | Tags: Drug Discovery
@article{Korshunova2021,
title = {OpenChem: A Deep Learning Toolkit for Computational Chemistry and Drug Design},
author = {Maria Korshunova and Boris Ginsburg and Alexander Tropsha and Olexandr Isayev},
doi = {10.1021/acs.jcim.0c00971},
issn = {1549-960X},
year = {2021},
date = {2021-01-25},
urldate = {2021-01-25},
journal = {J. Chem. Inf. Model.},
volume = {61},
number = {1},
pages = {7--13},
publisher = {American Chemical Society (ACS)},
abstract = {Deep learning models have demonstrated outstanding results in many data-rich areas of research, such as computer vision and natural language processing. Currently, there is a rise of deep learning in computational chemistry and materials informatics, where deep learning could be effectively applied in modeling the relationship between chemical structures and their properties. With the immense growth of chemical and materials data, deep learning models can begin to outperform conventional machine learning techniques such as random forest, support vector machines, and nearest neighbor. Herein, we introduce OpenChem, a PyTorch-based deep learning toolkit for computational chemistry and drug design. OpenChem offers easy and fast model development, modular software design, and several data preprocessing modules. It is freely available via the GitHub repository.},
keywords = {Drug Discovery},
pubstate = {published},
tppubtype = {article}
}
2020
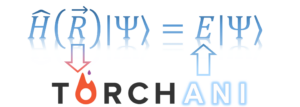
Gao, Xiang; Ramezanghorbani, Farhad; Isayev, Olexandr; Smith, Justin S.; Roitberg, Adrian E.
TorchANI: A Free and Open Source PyTorch-Based Deep Learning Implementation of the ANI Neural Network Potentials Journal Article
In: J. Chem. Inf. Model., vol. 60, no. 7, pp. 3408–3415, 2020.
Links | BibTeX | Tags: ANI, Machine learning potential
@article{Gao2020,
title = {TorchANI: A Free and Open Source PyTorch-Based Deep Learning Implementation of the ANI Neural Network Potentials},
author = {Xiang Gao and Farhad Ramezanghorbani and Olexandr Isayev and Justin S. Smith and Adrian E. Roitberg},
doi = {10.1021/acs.jcim.0c00451},
year = {2020},
date = {2020-07-27},
urldate = {2020-07-27},
journal = {J. Chem. Inf. Model.},
volume = {60},
number = {7},
pages = {3408--3415},
publisher = {American Chemical Society (ACS)},
keywords = {ANI, Machine learning potential},
pubstate = {published},
tppubtype = {article}
}
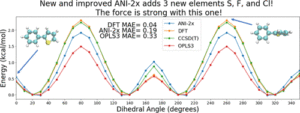
Devereux, Christian; Smith, Justin S.; Huddleston, Kate K.; Barros, Kipton; Zubatyuk, Roman; Isayev, Olexandr; Roitberg, Adrian E.
Extending the Applicability of the ANI Deep Learning Molecular Potential to Sulfur and Halogens Journal Article
In: J. Chem. Theory Comput., vol. 16, no. 7, pp. 4192–4202, 2020, ISSN: 1549-9626.
Abstract | Links | BibTeX | Tags: ANI, Machine learning potential
@article{Devereux2020,
title = {Extending the Applicability of the ANI Deep Learning Molecular Potential to Sulfur and Halogens},
author = {Christian Devereux and Justin S. Smith and Kate K. Huddleston and Kipton Barros and Roman Zubatyuk and Olexandr Isayev and Adrian E. Roitberg},
doi = {10.1021/acs.jctc.0c00121},
issn = {1549-9626},
year = {2020},
date = {2020-07-14},
urldate = {2020-07-14},
journal = {J. Chem. Theory Comput.},
volume = {16},
number = {7},
pages = {4192--4202},
publisher = {American Chemical Society (ACS)},
abstract = {Machine learning (ML) methods have become powerful, predictive tools in a wide range of applications, such as facial recognition and autonomous vehicles. In the sciences, computational chemists and physicists have been using ML for the prediction of physical phenomena, such as atomistic potential energy surfaces and reaction pathways. Transferable ML potentials, such as ANI-1x, have been developed with the goal of accurately simulating organic molecules containing the chemical elements H, C, N, and O. Here, we provide an extension of the ANI-1x model. The new model, dubbed ANI-2x, is trained to three additional chemical elements: S, F, and Cl. Additionally, ANI-2x underwent torsional refinement training to better predict molecular torsion profiles. These new features open a wide range of new applications within organic chemistry and drug development. These seven elements (H, C, N, O, F, Cl, and S) make up ∼90% of drug-like molecules. To show that these additions do not sacrifice accuracy, we have tested this model across a range of organic molecules and applications, including the COMP6 benchmark, dihedral rotations, conformer scoring, and nonbonded interactions. ANI-2x is shown to accurately predict molecular energies compared to density functional theory with a ∼106 factor speedup and a negligible slowdown compared to ANI-1x and shows subchemical accuracy across most of the COMP6 benchmark. The resulting model is a valuable tool for drug development which can potentially replace both quantum calculations and classical force fields for a myriad of applications.},
keywords = {ANI, Machine learning potential},
pubstate = {published},
tppubtype = {article}
}
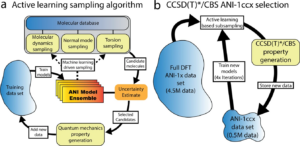
Smith, Justin S.; Zubatyuk, Roman; Nebgen, Benjamin; Lubbers, Nicholas; Barros, Kipton; Roitberg, Adrian E.; Isayev, Olexandr; Tretiak, Sergei
The ANI-1ccx and ANI-1x data sets, coupled-cluster and density functional theory properties for molecules Journal Article
In: Sci Data, vol. 7, no. 1, 2020, ISSN: 2052-4463.
Abstract | Links | BibTeX | Tags: ANI, dataset, Machine learning potential
@article{Smith2020,
title = {The ANI-1ccx and ANI-1x data sets, coupled-cluster and density functional theory properties for molecules},
author = {Justin S. Smith and Roman Zubatyuk and Benjamin Nebgen and Nicholas Lubbers and Kipton Barros and Adrian E. Roitberg and Olexandr Isayev and Sergei Tretiak},
doi = {10.1038/s41597-020-0473-z},
issn = {2052-4463},
year = {2020},
date = {2020-01-01},
urldate = {2020-01-01},
journal = {Sci Data},
volume = {7},
number = {1},
publisher = {Springer Science and Business Media LLC},
abstract = {Maximum diversification of data is a central theme in building generalized and accurate machine learning (ML) models. In chemistry, ML has been used to develop models for predicting molecular properties, for example quantum mechanics (QM) calculated potential energy surfaces and atomic charge models. The ANI-1x and ANI-1ccx ML-based general-purpose potentials for organic molecules were developed through active learning; an automated data diversification process. Here, we describe the ANI-1x and ANI-1ccx data sets. To demonstrate data diversity, we visualize it with a dimensionality reduction scheme, and contrast against existing data sets. The ANI-1x data set contains multiple QM properties from 5 M density functional theory calculations, while the ANI-1ccx data set contains 500 k data points obtained with an accurate CCSD(T)/CBS extrapolation. Approximately 14 million CPU core-hours were expended to generate this data. Multiple QM calculated properties for the chemical elements C, H, N, and O are provided: energies, atomic forces, multipole moments, atomic charges, etc. We provide this data to the community to aid research and development of ML models for chemistry.},
keywords = {ANI, dataset, Machine learning potential},
pubstate = {published},
tppubtype = {article}
}
2017
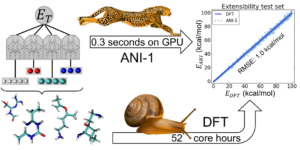
Smith, Justin S.; Isayev, Olexandr; Roitberg, Adrian E.
ANI-1: an extensible neural network potential with DFT accuracy at force field computational cost Journal Article
In: Chemical Science, iss. 8, pp. 3192-3203, 2017.
Abstract | Links | BibTeX | Tags: ANI, Machine learning potential
@article{Smith2017,
title = {ANI-1: an extensible neural network potential with DFT accuracy at force field computational cost},
author = {Justin S. Smith and Olexandr Isayev and Adrian E. Roitberg },
url = {https://olexandrisayev.com/wp-content/uploads/2024/02/c6sc05720a.pdf},
doi = {10.1039/C6SC05720A},
year = {2017},
date = {2017-02-08},
urldate = {2017-02-08},
journal = {Chemical Science},
issue = {8},
pages = {3192-3203},
abstract = {Deep learning is revolutionizing many areas of science and technology, especially image, text, and speech recognition. In this paper, we demonstrate how a deep neural network (NN) trained on quantum mechanical (QM) DFT calculations can learn an accurate and transferable potential for organic molecules. We introduce ANAKIN-ME (Accurate NeurAl networK engINe for Molecular Energies) or ANI for short. ANI is a new method designed with the intent of developing transferable neural network potentials that utilize a highly-modified version of the Behler and Parrinello symmetry functions to build single-atom atomic environment vectors (AEV) as a molecular representation. AEVs provide the ability to train neural networks to data that spans both configurational and conformational space, a feat not previously accomplished on this scale. We utilized ANI to build a potential called ANI-1, which was trained on a subset of the GDB databases with up to 8 heavy atoms in order to predict total energies for organic molecules containing four atom types: H, C, N, and O. To obtain an accelerated but physically relevant sampling of molecular potential surfaces, we also proposed a Normal Mode Sampling (NMS) method for generating molecular conformations. Through a series of case studies, we show that ANI-1 is chemically accurate compared to reference DFT calculations on much larger molecular systems (up to 54 atoms) than those included in the training data set.},
keywords = {ANI, Machine learning potential},
pubstate = {published},
tppubtype = {article}
}