Applied generative modeling offers an opportunity to reformulate design and discovery in the chemical sciences. Examples of generative models that suggest unexplored chemical species are now abundant. I believe it is time to look beyond demonstrating the operability of generative models and move toward their practical implementation for solving scientific challenges.
Arguably, a holy grail of modern chemical research is the implementation of an efficient closed-loop discovery process, where target molecules or materials are generated, synthesized, characterized, and refined with minimal human input. This can be in the form of self-driving experimentation or autonomous quantum mechanical calculations followed by synthetic chemistry, to name a few. Below are several examples of Generative AI works from my lab.
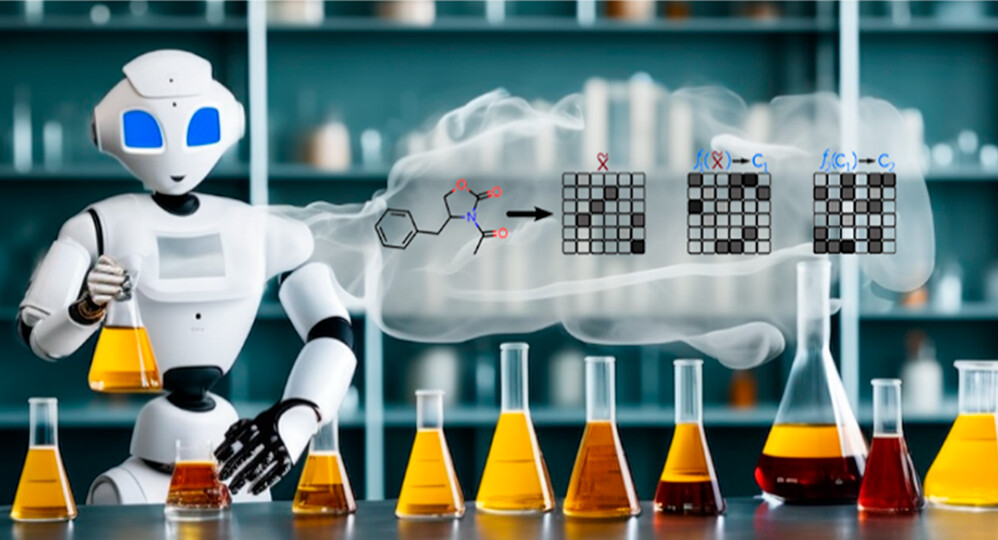
Dylan M. Anstine, Olexandr Isayev. Generative Models as an Emerging Paradigm in the Chemical Sciences. J. Am. Chem. Soc., 2023, 145, 16, 8736–8750. DOI: 10.1021/jacs.2c13467.
Automated de novo design of bioactive compounds
In the context of molecule or material discovery, actions can be thought of as selecting functional motifs, sequentially building chemical structures, or autoregressive species construction, to name a few. The selection of molecule or material-building actions based on the current state of the system is referred to as the policy. See our methodological paper: Deep reinforcement learning for de novo drug design.
Following the generation of a complete SMILES string, a reward is given in accordance with the perceived value the molecule possesses for the target application. In policy gradient methods, i.e., those aimed at refining action selection, the reward signal determines the model parameter updates and, therefore, is responsible for guiding future actions. Most RL frameworks operate with the goal of maximizing the cumulative sum of rewards. For generative models aimed at discovery, an RL system seeks to leverage accumulated experience of testing molecules and materials for a target purpose to build better species with each training cycle.
In a proof-of-concept study, we demonstrated the application of the deep generative recurrent neural network architecture enhanced by several proposed technical tricks to design inhibitors of the epidermal growth factor (EGFR) and further experimentally validate their potency.
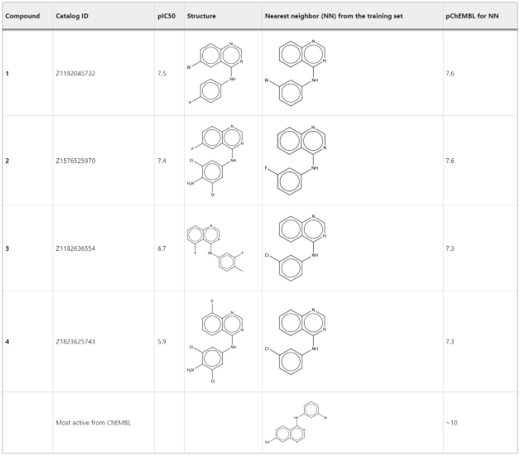
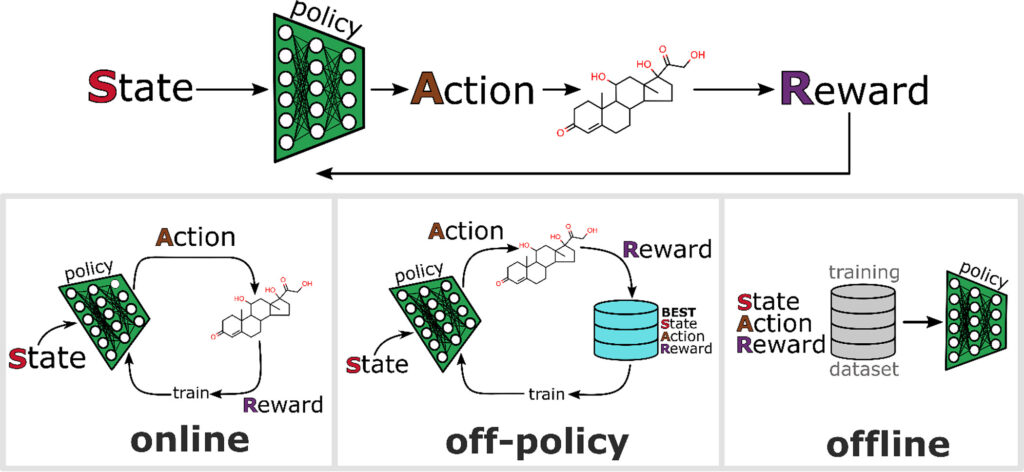
Illustration of reinforcement learning (RL) as an approach to generate chemical species. Training strategies include online, off-policy, and offline. In online RL, the most up-to-date policy is used to generate molecules. Off-policy RL carries out policy updates using a stored collection of best states, actions, and rewards. In offline RL, the training data set is external, and the policy optimization is akin to a traditional supervised learning problem.
Korshunova, M., Huang, N., Capuzzi, S. et al. Generative and reinforcement learning approaches for the automated de novo design of bioactive compounds. Commun Chem,. 2022, 5, 129. DOI: 10.1038/s42004-022-00733-0.
Out of fifteen tested compounds that were predicted active, four were confirmed in an EGFR enzyme assay. Two out of four compounds had nanomolar EGFR inhibition activity comparable to that of staurosporine. The overall hit rate was ~27%. Additionally, five compounds with the same scaffold as in active compounds but predicted as inactive were used as a negative control. All five compounds were confirmed as inactive.
The obtained hit rate is on par with traditional virtual screening projects where molecule selection is guided by an expert medicinal chemist. However, in this work, we show that a properly trained AI model can mimic medicinal chemists’ skills in the autonomous generation of new chemical entities (NCEs) and selection of molecules for experimental validation. This is a prime example of the transfer of the decision power from human experts to AI. Such capabilities could be an important step toward true self-driving laboratories and serve as an example of the synergy between machine and human intelligence.