In our latest paper, we present the 2nd generation of our atoms-in-molecules neural network potential (AIMNet2), which is applicable to species composed of up to 14 chemical elements in both neutral and charged states, making it a valuable model for modeling the majority of non-metallic compounds. Using an exhaustive dataset of 20 million hybrid quantum chemical calculations, AIMNet2 combines ML-parameterized short-range and physics-based long-range terms to attain generalizability that reaches from simple organics to diverse molecules with “exotic” element-organic bonding. We show that AIMNet2 outperforms semi-empirical GFN-xTB and is on par with reference density functional theory for interaction energy contributions, conformer search tasks, torsion rotation profiles, and molecular-to-macromolecular geometry optimization. Overall, the demonstrated chemical coverage and computational efficiency of AIMNet2 are significant steps toward providing access to MLIPs that avoid the crucial limitation of curating additional quantum chemical data and retraining with each new application.
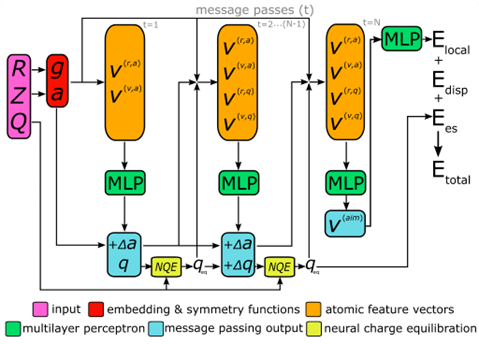
A schematic overview of the AIMNet2 architecture is shown above. AIMNet2 calculates the total energy of a chemical system according to:
Etotal = Elocal + Edisp + Ees
where Elocal, Edisp, and Ees refer to the local configurational interaction energy, explicit dispersion correction, and electrostatics between atom-centered partial point charges, respectively. Similar to the previous version of AIMNet, multi-task predictions can be constructed on-top of the learned representation, i.e., the so-called AIM vector (), but we chose to omit them for clarity. However, this feature supports the flexibility of AIMNet2 to be applied to diverse molecular and material systems because the functional form can be readily tailored to meet the demands of the modeling task by including additional output heads. We include explicit dispersion interactions using a PyTorch implementation of the DFT-D3 correction model. All source code and pretrained models used in this work are provided in the open-source repository https://github.com/isayevlab/AIMNet2 on GitHub.